This April, I gave a talk at ETE 2024 called “The Tools We Still Need to Build with AI.”
I think people expected me to list my predictions for profitable shovels to sell in the AI1 gold rush. I’ve been accused of playing 4-D chess: baiting the audience who needs to hear this and switching in what they need to hear. I wish I could claim that devious chutzpah. I can’t.
I didn’t intend to mislead anyone; I learned a lot this spring about the difference between pitching inside a very sexy topic and pitching the uninspiring drivel I’m used to. You know: technical debt. Or debugging. Or feedback.
To some of the ETE audience’s dismay, I never recommended any specific AI product to buy or build. Instead, I offered an interpretation of tech history that I believe could—maybe even should—inform the work of someone who might buy or build AI stuff. I believe there’s a pattern to be observed in the development of successful tech products, and that pattern has something to tell us about emergent platforms like, arguably, generative models.
I’m not hearing good analysis on this from folks whose enthusiasm for generative technology overtakes their willingness to think critically about it. I give workshops on generative technologies for O’Reilly. We frequently spend an appreciable amount of time discussing the fear of LLMs taking over peoples’ jobs. I wouldn’t put my University students in the “enthusiasm” category—I think their desire to use LLMs hinges less on unbridled excitement and more on coercion from the tech zeitgeist—but it produces the same questions.
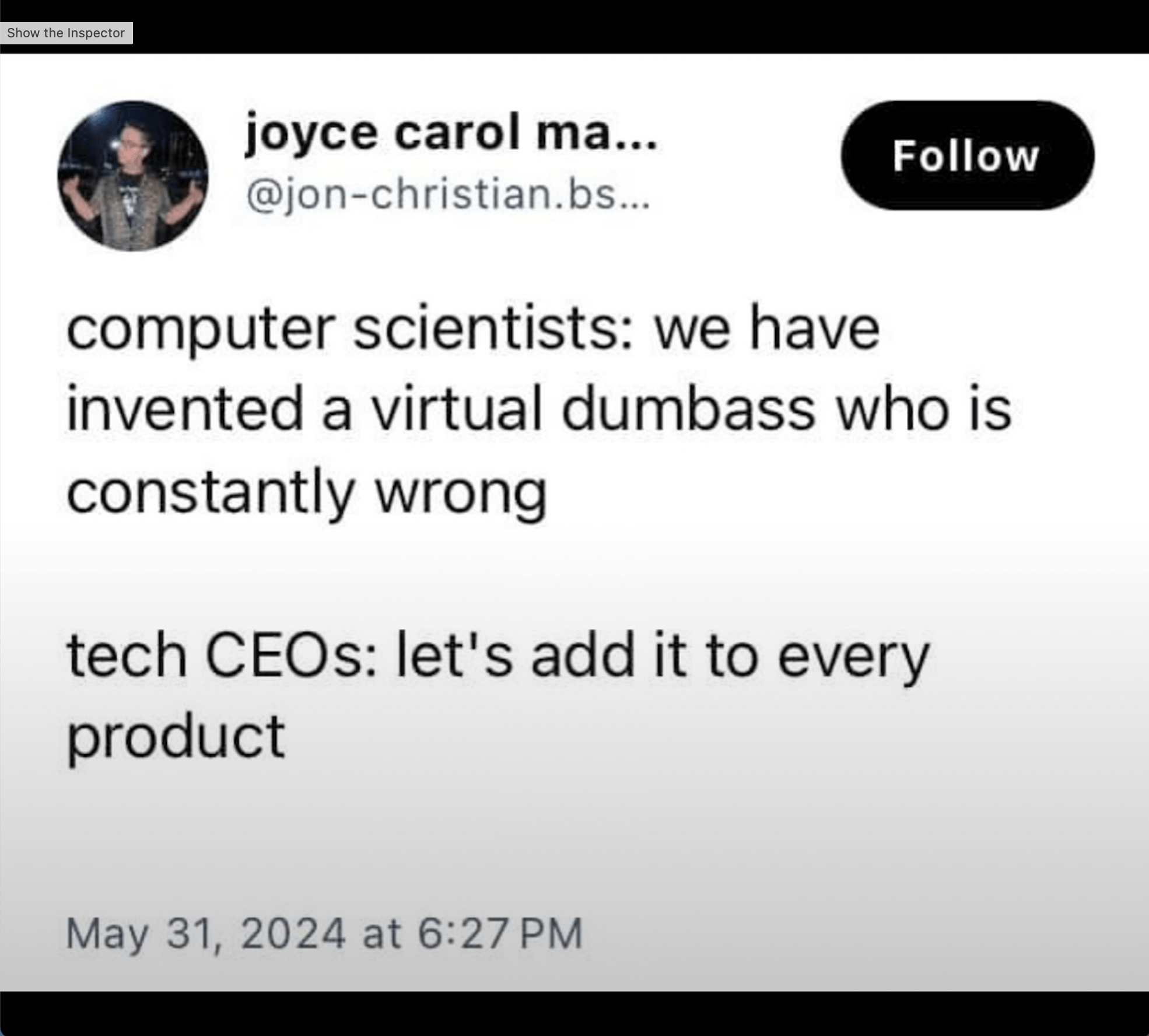
I don’t think that AI doomsdayism has a lot to offer us on these questions, either. Those circles repeatedly insist that what we need to do now is throw the whole thing away, or regulate it immediately. Though I agree that regulation should carry more priority in tech in general (not just AI), it hasn’t happened in all of commercial software’s 742 year history, and anti-regulation interests have never possessed a stronger chokehold on the tech boardroom or the legislative chamber than they do today. Not to mention, even if regulation happened this evening, we’d still be left with all of the questions of enforcement and effectiveness that we face in already regulated endeavors. Generative AI products have reached a level of ubiquity that would make them difficult to yank off the market and stuff back into a jar. So I think technologists themselves (and, for better or worse, the management consultants in their employers’ executive roles) carry a lot of responsibility to build the tools that put us in the AI future we want to create.
And preparing for that means we gotta talk about exactly the sort of unsexy shit I traffic in: today, it’s history!
And specifically, what we can learn from the patterns of commercial tech platforms that came before the AI spring. I’m hopeful that patterns from our past can help me sketch out a framework for you to conceptualize product innovation in the tech space at the dawn of a sea shift in the availability of a substrate consumer technology.
SCENE: The 1990s. Dawn of the Consumer-Oriented Web Browser.
Products and companies that we remember from those first ten years of e-commerce often came from college dudes. Remember this? These were the Ebay days. The early Amazon and Google days.
The college dudes dudes were almost always wealthy and well-connected, but they weren’t usually market-savvy.
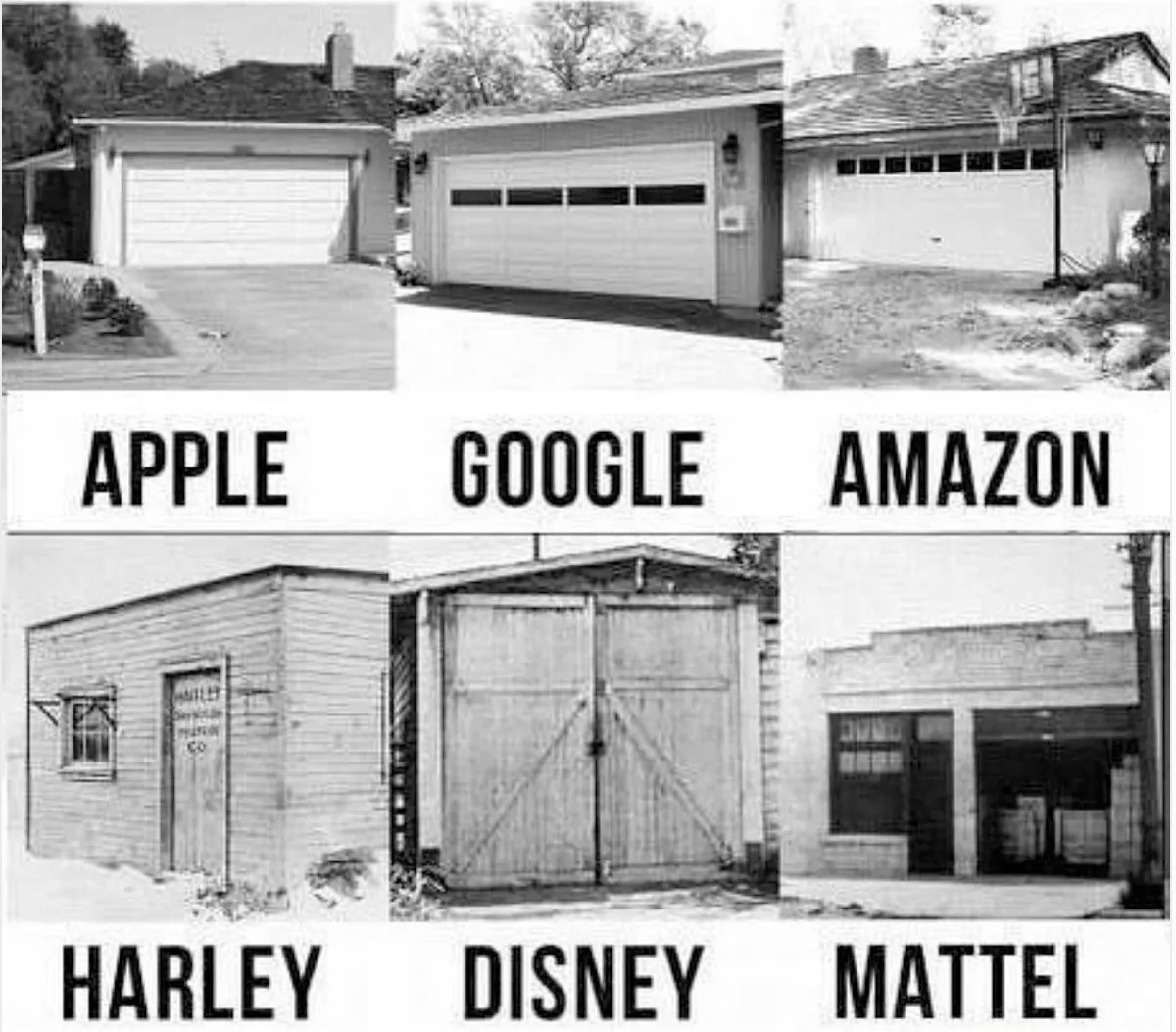
These people, now billionaires, have given lots of interviews on how they got started. They all say “I made a thing I wanted to use. Other people liked it. That was literally it.”
In the book How the Internet Happened, author Brian McCullough relays the story of Pierre Omidyar talking about the founding of ebay. At that time you could absolutely buy things on the internet, but you’d, like, go to some random guy’s website to buy a copy of a VHS tape. Picture the online equivalent of walking up to a dude in a parking lot and buying that same VHS tape out of the trunk of his sedan. Not a fake example: it’s how Rand Gauthier sold the personal footage of Tommy Lee and Pamela Anderson that he stole form their home circa 1996. That scandal concisely characterized proto-e-commerce’s contemporary reputation: seedy, unreliable, of questionable legality. In a word: sketchy.
Pierre started ebay as an experiment on his personal website. That product, with that timing, paved the way for the customer rating: an opportunity for the crowd to grade sellers on their reliability and product quality in the absence of formally oriented regulation structures (which I’d argue, for the internet, still haven’t materialized—instead Amazon ratings, Yelp, and other crowd-soliciting products commercially answer this need with patchy success). Back to Pierre at ebay: he didn’t start with a bunch of consumer research. He played with a thing on his personal website, and it took off.
How often do you suppose tech unicorns today come from some college dude independently thinking up an idea? Today, tech companies—particularly large ones—approach product development pretty differently to that. The modern professional product manager rarely builds based on whims, curiosity, and pure unadulterated self-interest. They instead want to be “data-driven.” They focus on quantitative methods to find product-market fit. They interview people who use the product or survey people in majority groups that they would like to see using the product. They also regularly triangulate information from product surveillance data.
Born of the peer-to-peer selling boom with ebay as the flagship example, customer reviews now have whole sub-industries around them. In 2024, technical book publishers3 choose what titles to release based on which titles from market leaders sell the most copies on Amazon. Then, they’ll trawl the reviews of those books to look for information about what the books lacked, and coach their own authors to fill in those gaps.
Product distributors know that their customer reviews matter, so there’s also a cottage industry around hiring folks to give reviews, or even better, spinning up bots to give many good reviews. Then, of course, you have your counter-industry for separating the real reviews from the fake ones.
“Build for yourself” worked for founders in the nineties, but now gets cast as immature and shortsighted. Now, you need data.
What changed?
Understanding what changed reveals a false dichotomy between “build for yourself”
and “build based on your focus group data.” Because declaring that ebay won due to timing simplifies this story way too much; there are plenty of other ways besides “bad timing” to torpedo your chances at product success.
In 2021, Slack built direct messaging across Slack instances. It was in production before anybody (anybody being, of course, the entire internet) pointed out that the feature could hardly cater to harassers and stalkers more perfectly. If this misuse case could become so apparent so quickly the moment the feature hit the market, how did Slack fail to catch it before investing in the feature in the first place?
What happens is, companies collect data and then focus on the highest ranked outcome or the majority outcome. Keeping focus on the center can lead organizations to efforts that don’t innovate, and sometimes don’t even work.
In the fall of 2020, Americans protested the curtailment of the U.S. Postal Service favor of private options like FedEx or UPS. Wealthy folks in populous areas don’t realize the difference between the USPS and the private options, but it’s a great example of the difference between government policymaking and standard business decision-making. The reason the USPS matters so much is that the USPS delivers everywhere. As in, a postal worker mounts a mule to deliver letters to the Havasupai people at the bottom of the Grand Canyon.
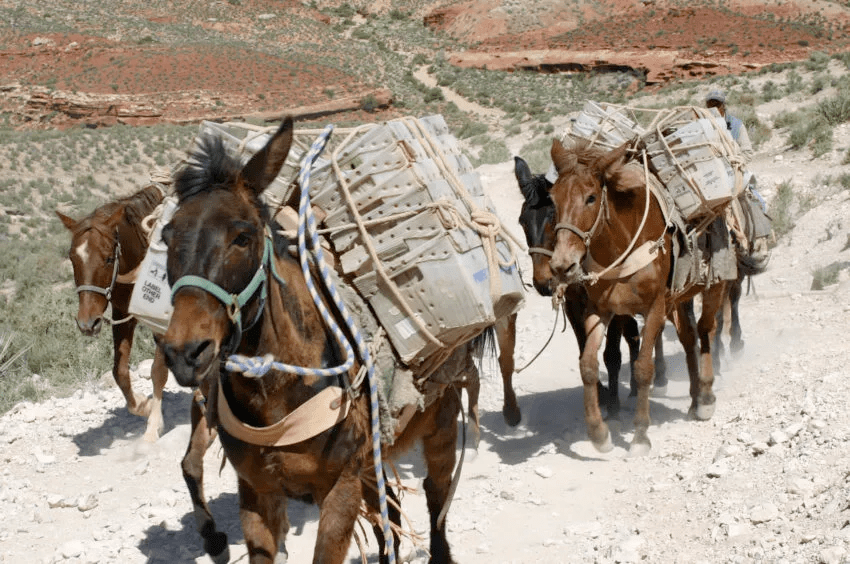
Businesses often tend to focus on efficiencies—doing the things that net them the most money for the least effort. By contrast, taxpayer-funded public programs are designed and expected to cover everyone—including, and especially, the most marginalized. That’s why they’re taxpayer-funded; so they don’t face existential risk be eschewing profit-driven decision-making.
Does this work perfectly? No. But I think about it a lot when people kvetch about the bigness and slowness of government. That bigness and slowness is supposed to create space and resources to account for the communities that a “lean” approach deliberately ignores.
Folks tend to argue that businesses are profit-driven, and therefore HAVE to do things this way. I think executives default to the decision-making strategies to which they’ve become accustomed; that doesn’t mean they have to do it, and it doesn’t mean they should.
To understand why not, let’s return to the Slack connect example. That product had to be delayed for redesign and then re-released with major changes. Do you know how much that costs? Had the design process included a marginalized, abuse-informed opinion, they might have enjoyed a much more cost-effective market entry.
Or take Google’s decision in 2020 to withhold Timnit Gebru’s work on their Ethical AI team. She and three other researchers at Google participated in co-authorship of a paper called “On the Dangers of Stochastic Parrots: Can Language Models Be Too Big?”
The paper pointed out:
- the carbon footprint of training large language models
- the biases and prejudices they could recreate from patterns in training data
- the imbalance in training data toward the languages and linguistic norms of rich, highly online cultures
- the homogenizing effect that the resulting models could have on language and culture
- the degree to which the illusion of meaning of the language coming out of a convincing language generator could be used to generate and spread misinformation or deliberately mislead
Google took issue, they said, with the methodological rigor of the paper; other Googlers counterargued that the company had never launched such an inquisition of their literature reviews as this one. Dr. Gebru set an ultimatum, and Google AI lead Jeff Dean used it to justify Dr. Gebru’s termination while she was out of office. Margaret Mitchell, Dr. Gebru’s co-lead, also got fired two months later. The reason, depending on who you ask, varies wildly.
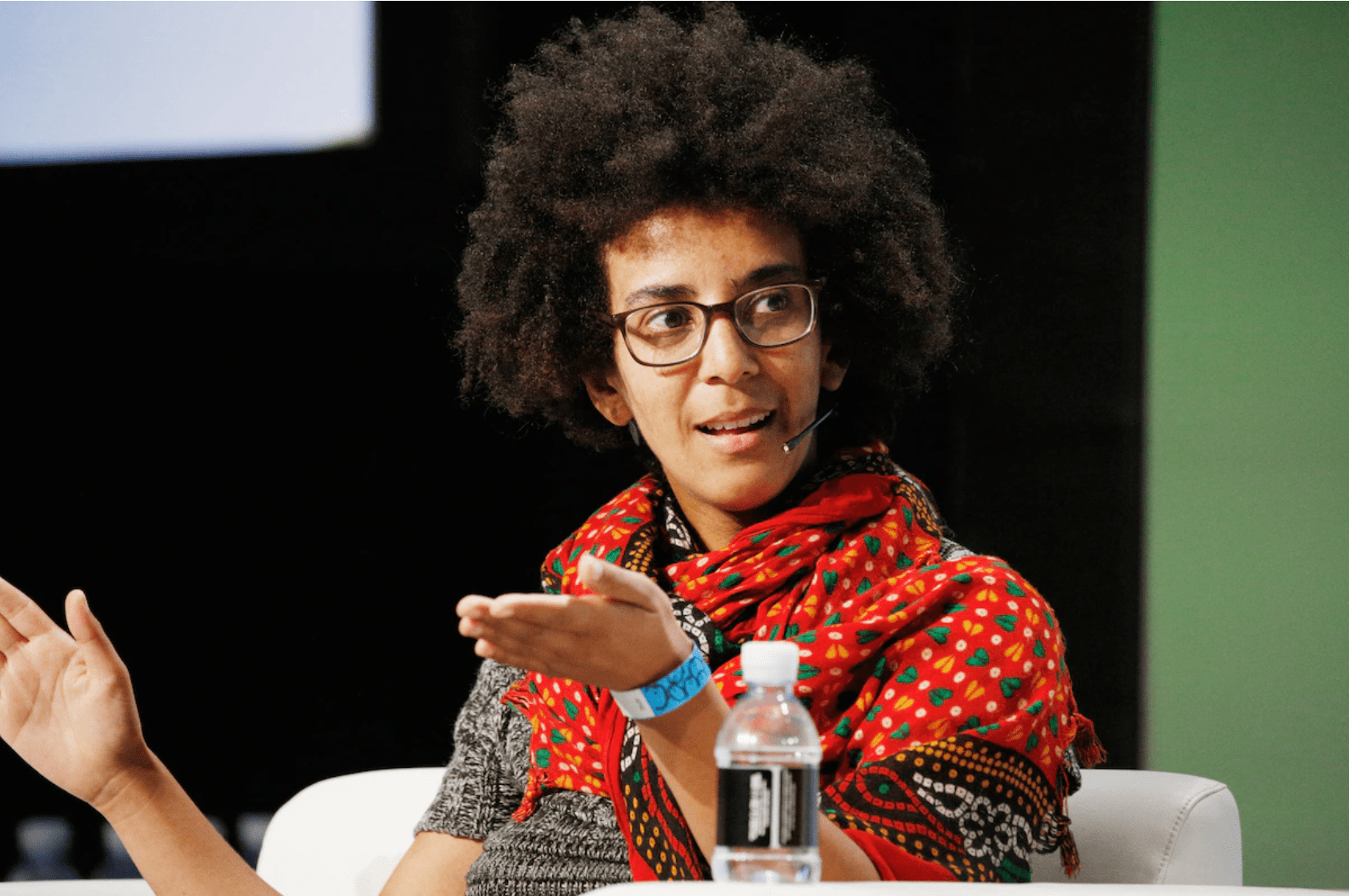
The trust that Google lost from that event came with a price tag. Companies that lose the trust of hiring prospects have to work harder to recruit. They have to compensate higher to get their offers accepted. And the folks best positioned to drive visionary product strategy in the AI space aren’t going to go there at all. I’m not saying this is why Gemini, Google’s LLM product, lags remarkably far behind ChatGPT in terms of customer satisfaction. I can think of a few additional reasons, not all of which incriminate Google4. Nevertheless, companies can’t be shocked by lackluster outcomes after sending signals that affect their ability to hire.
You might argue to me that a couple of failures at companies that ignored the margins doesn’t prove anything, and that’s fair. When has centering a marginalized perspective produced success and riches for a company?
In 2007, we got the iPhone.
Steve Jobs himself presented the iPhone at MacWorld that year, and the story of that presentation has become part of tech industry canon. Because the iPhone wasn’t just a product. The iPhone was a different thing entirely: a platform. A place where other people and other companies could eventually build their own mobile applications. The iPhone launch isn’t comparable to Ebay or Napster or Amazon’s original book sale website. The iPhone, by being a platform, changed the tech industry the same way the commercial internet itself did. It didn’t just enter a competitor into the space. It created a whole new product arena in which techies could compete.
How did Apple manage to do this? People loooove the iPhone as an example of visionary success for a tech company. And I love it because everybody uses this example while completely miscontextualizing it.
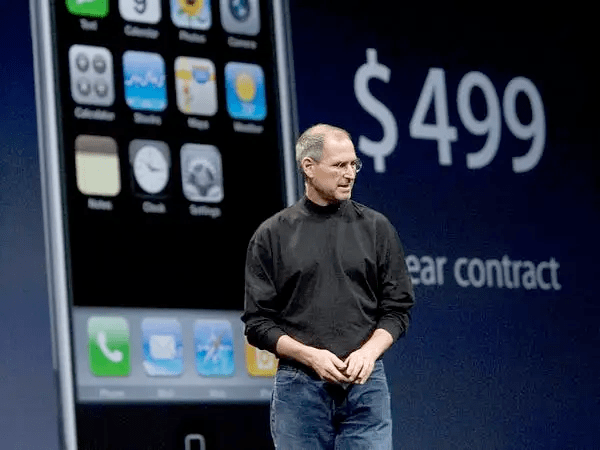
What made the iPhone a platform rather than just a product? Most famously, the iPhone’s capacitative multi-touch screen. That screen—unlike the tiny keyboards on other mobile devices of the day—made it possible to dream up any user interface design. That feature enabled the iPhone’s ascendance to the status of a platform for independent application development. It broke the market for existing phones.
Where did that screen come from? Turns out, Steve Jobs wasn’t some kind of genius visionary who independently thought that thing up. Its roots came from FingerWorks, an accessible human-computer interaction company founded with the express purpose of creating input devices for folks with compromised fine motor function. One of the founders wanted to build a way for his disabled mom to continue doing all the things she wanted to do, and that grew into the company that Apple bought in 2005.
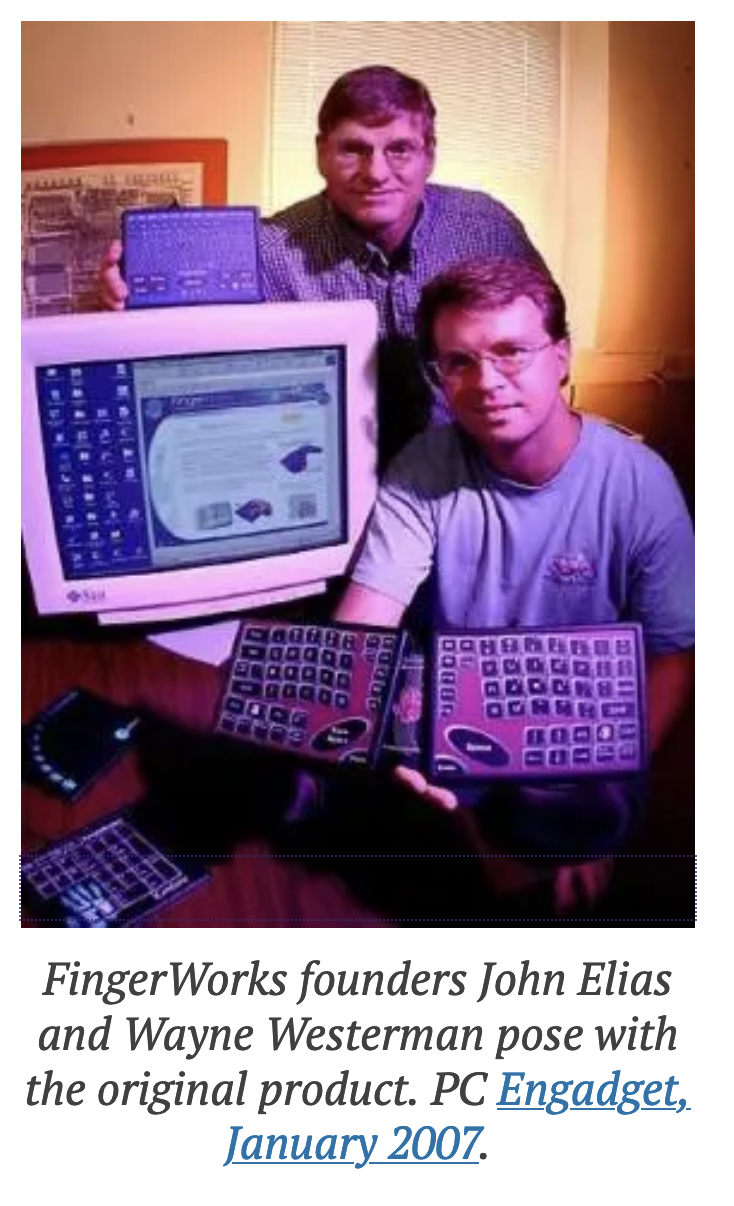
It wasn’t Apple’s idea; Steve had to be cunningly convinced, through a whole lattice of coordinated strategies, to put the thing in the iPhone at all. In fact, most of the things you love about your smartphone started as accessibility features.
I told you earlier that we’d come back to an idea: garden variety Pareto Principle-style business thinking doesn’t work for visionary product development. Companies can incur costly failures by mis-applying it. Companies can also achieve momentous victories by approaching product development questions differently. Designing for the edges can produce something better for everyone.
But what does this have to do with data-driven product decisions, and how we got to those from “build for yourself?”
Well, let’s summarize what we’ve learned so far:
- Building for the majority group identified in summary statistics doesn’t produce
visionary decisions. - Organizations can incur costly failures, and even product irrelevance, by
doing that. - Organizations can achieve momentous victories by
prioritizing marginal business cases.
The catch is that building for the majority group identified in summary statistics is
exactly the trap that data-driven decision making can fall into.
Back to the original question I started us down this tangent with:
“What changed in tech from the 1990s to now that influenced the switch from
well-positioned college kids building their dreams to dads in graphic tees analyzing
spreadsheets?”
What changed was who constituted the “marginal business case.” Stick with me here.
In the 1990s, personal computers as a mainstream thing were brand new. That’s
when we get the paradigm-shifting “visionary” internet successes like eBay, Google,
Apple, Amazon, and eventually Netflix. Netflix became kinda the youngest famous child of this particular generation of the commercial internet. Who did Netflix kill?
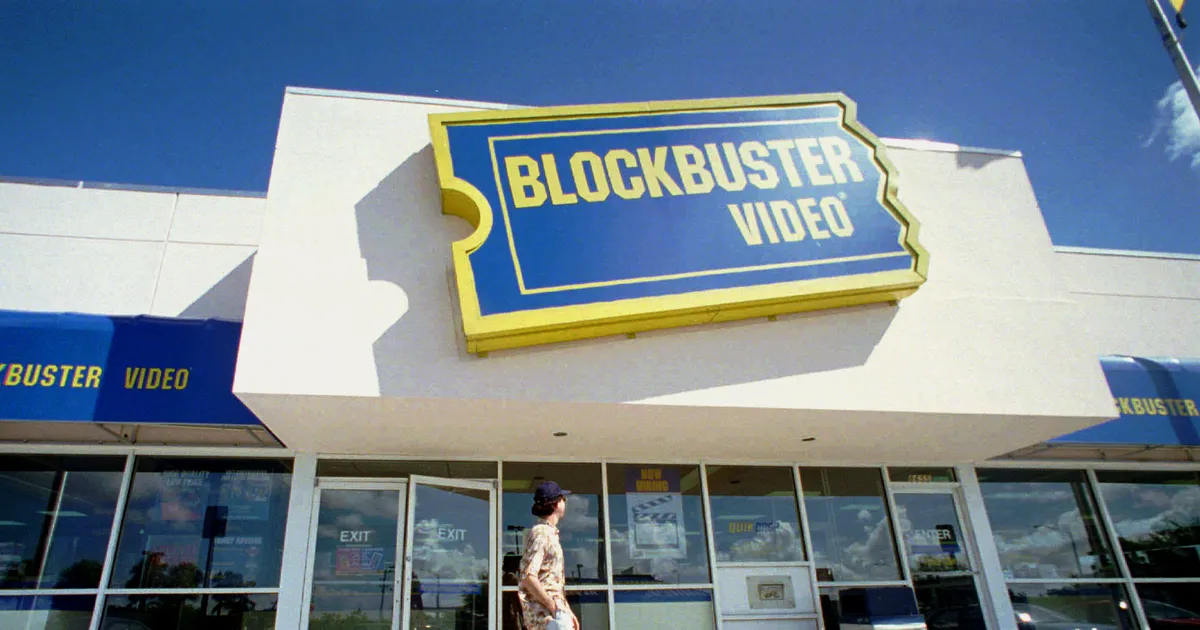
Blockbuster. Before Netflix, you went to Blockbuster to rent movies. You picked up a physical VHS tape, or DVD, and you took it home, and you brought it back before a specific due date or they’d charge you more money. Netflix didn’t start as a streaming service. It started as a service where you could go online and make a list of movies you wanted to see, and they’d mail you the DVD, and then when you were done you’d mail it back. It killed Blockbuster, and it eventually launched a race for competing services like Amazon Prime and Hulu and HBO Max. It wasn’t even a streaming service to start with, but it’s one of the companies we list when we list the modern tech titans: the FAANG. Facebook, Apple, Amazon, Netflix. Then Google.
Do you know where visionary ideas come from?
Let’s break this down, because I need you to see this.
We describe ideas as “visionary” when they break from majority logic to imagine a
completely different future—one that serves currently unserved needs or poorly
served needs in a completely new way.
Do you know who has unserved needs or poorly served needs?
Marginalized groups. People that the current systems ignore or rebuff.
Visionary ideas derive directly from centering people at the margins.
Now, what the heck does “centering people at the margins” have to do with wealthy, well connected college kids making stuff up for fun? To understand that, we need to talk about what marginalization meant in the 1990s when the consumer web, as a platform, was new. At that time, in situ “applications” like bookstores, post offices, auctions, and Blockbusters had a service gap: location dependence. You had to be at the place. That limited access to folks with time & transportation. It limited access even for wealthy, well-connected college kids.
The location of the wealthy, well-connected college kid founders might have exacerbated their experience of location marginalization in the ‘90s. See, the U.S. looked different then. The Big Stuff from a STEM perspective still largely happened in Boston or NYC. Silicon Valley was growing, but it wasn’t what it is now. In the 1950s and 1960s, electrical and mechanical engineers moved out west do hardware research—often for defense companies like Lockheed. Their kids grew up and became the Dorm Room Garage Billionaires.
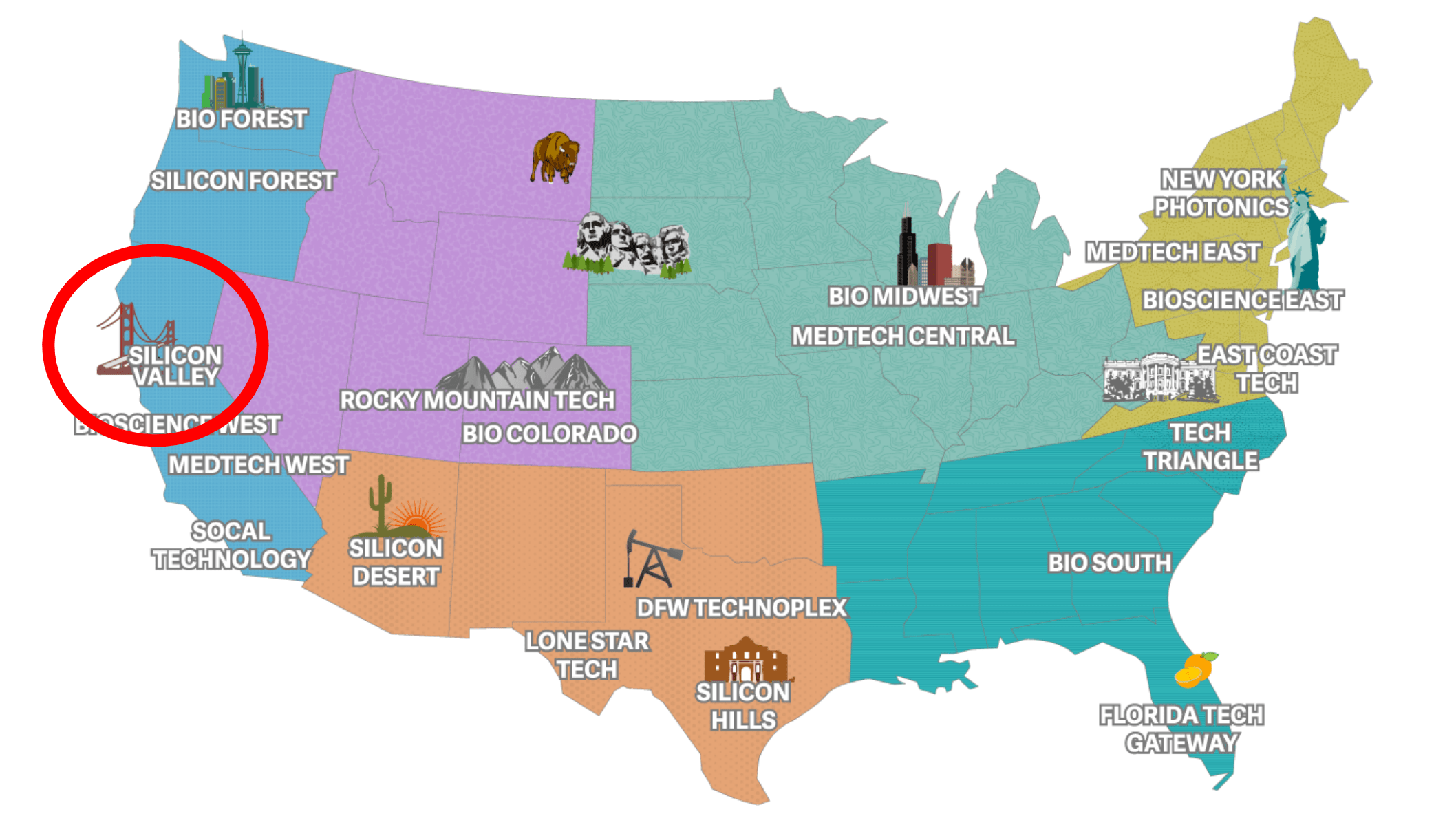
The Dorm Room Garage billionaires managed their riches and visionary status in large part because, for a glimpse of time, when the consumer web blinked into existence, those privileged kids experienced a marginalization—location marginalization—that the web suddenly made addressable. By building for themselves, in that glimpse of time, they centered a marginal need—to access resources and communities without co-temporal colocation.
For that glimpse of time, in other words, because everyone experienced a marginalization that this new platform—the web—addressed, even the most privileged person building for themselves was centering a marginalization. Was visionary.
Nowadays, of course, tech doesn’t look the same. We’ve had the commercial internet for 30 years. The dominant demographic has saturated the market with products they got to build for themselves. Building for already-centered people doesn’t produce visionary ideas. We see this in the patchy success from the secondary ventures of the same folks who built for themselves in that first generation: their most successful investments in the late aughts tended to either live in e-commercial logistics or in businesses that pursued an empirically confirmed demand for a product or service.
The others include, and look a lot like, Twitter: a company that generated enormous enthusiasm among investors but couldn’t stay cashflow positive. Tech has noticed this shift, but it doesn’t want to admit the whole well-connected rich dudes saturating the market part, so it has summarily discounted “build for yourself” as a product strategy instead.
And this, my friends, is the false dichotomy.
Product teams forget the context of the “build for yourself” spirit that they discount in favor of data-driven decisions. And this has tragically obscured their potential for actual visionary work.
Here’s why: It’s not “building for yourself doesn’t shift paradigms.”
It’s “building for yourself on a saturated platform doesn’t shift paradigms if you are already the main character.”
Technical product teams are overwhelmingly led by people who are already main characters in tech. So those main characters building for themselves produces incrementalist, weak willed, un-visionary work.
But!
Under those same circumstances, a data-driven approach that centers mainstream opinion also produces incrementalist, weak-willed, un-visionary work!
In a saturated market, achieving visionary product outcomes requires centering the still-marginal needs—which often means centering marginalized people and addressing their concerns.
Over the last 30 years, we’ve watched the commercial internet platform nurture three generations of product development.
The first generation—when the consumer internet was new—got to build for themselves with the confidence that the platform itself addressed a marginalization that everyone was feeling, so their products captured the enthusiasm and the dollars of the public. Surrounding and following the release of Netscape Navigator, the first web browser for the masses, we see the launch of companies like Amazon, Ebay, Google, and Netflix.
The second generation—when the consumer web got saturated—had to look for smaller crevices to fill, and found some by looking to data, because the public got to identify the opportunities that the garage dudes had missed. Netflix captured on an early one, with its release of a video streaming service in 2007. We got Twitter in 2006 and Slack in 2009—all companies with significant contributions to the blogosphere on the topic of data-driven product decisions.
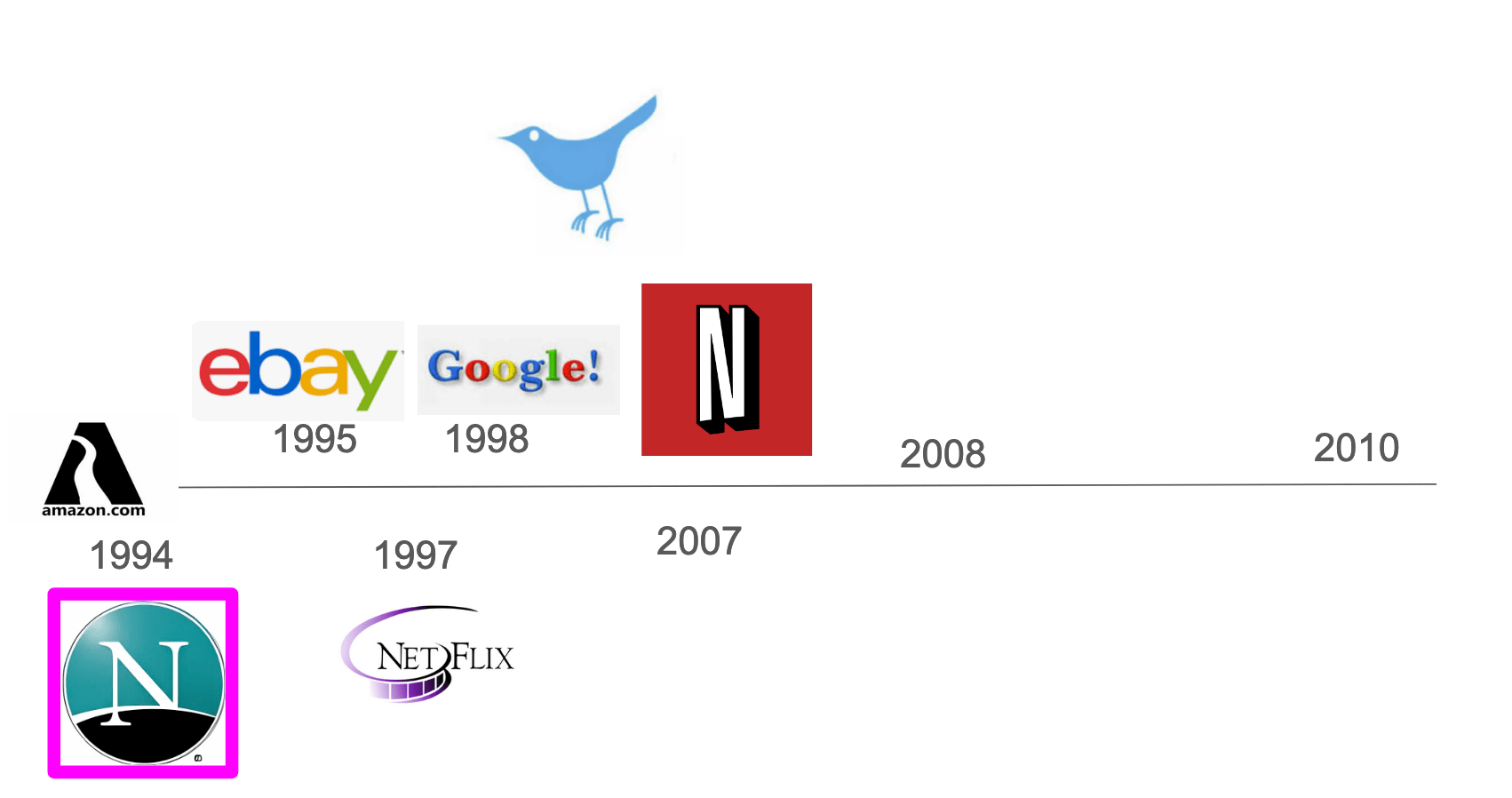
The third generation—the hardest generation to succeed in by clinging to mainstream and mediocrity, but the one from which the biggest innovations burst—requires us to find a need that the current platform either cannot address or has not bothered to address. We’re firmly in this territory of platform maturity with the consumer web, at this point.
I think we can argue that the iPhone introduced another platform, with its own
generations of product development.
It did this thanks to hardware innovations it acquired from folks who wanted to address a marginalized accessibility need. Accessibility solutions, though often uninspiring to executives, have a track record of amassing broad popularity once released.
A year after that MacWorld, in 2008, Apple released the app store, and then anyone could make a mobile app. The top apps from 2009 included Google maps and Amazon Kindle—both products from established tech companies that had data suggesting these things would be popular on the pocket computer.
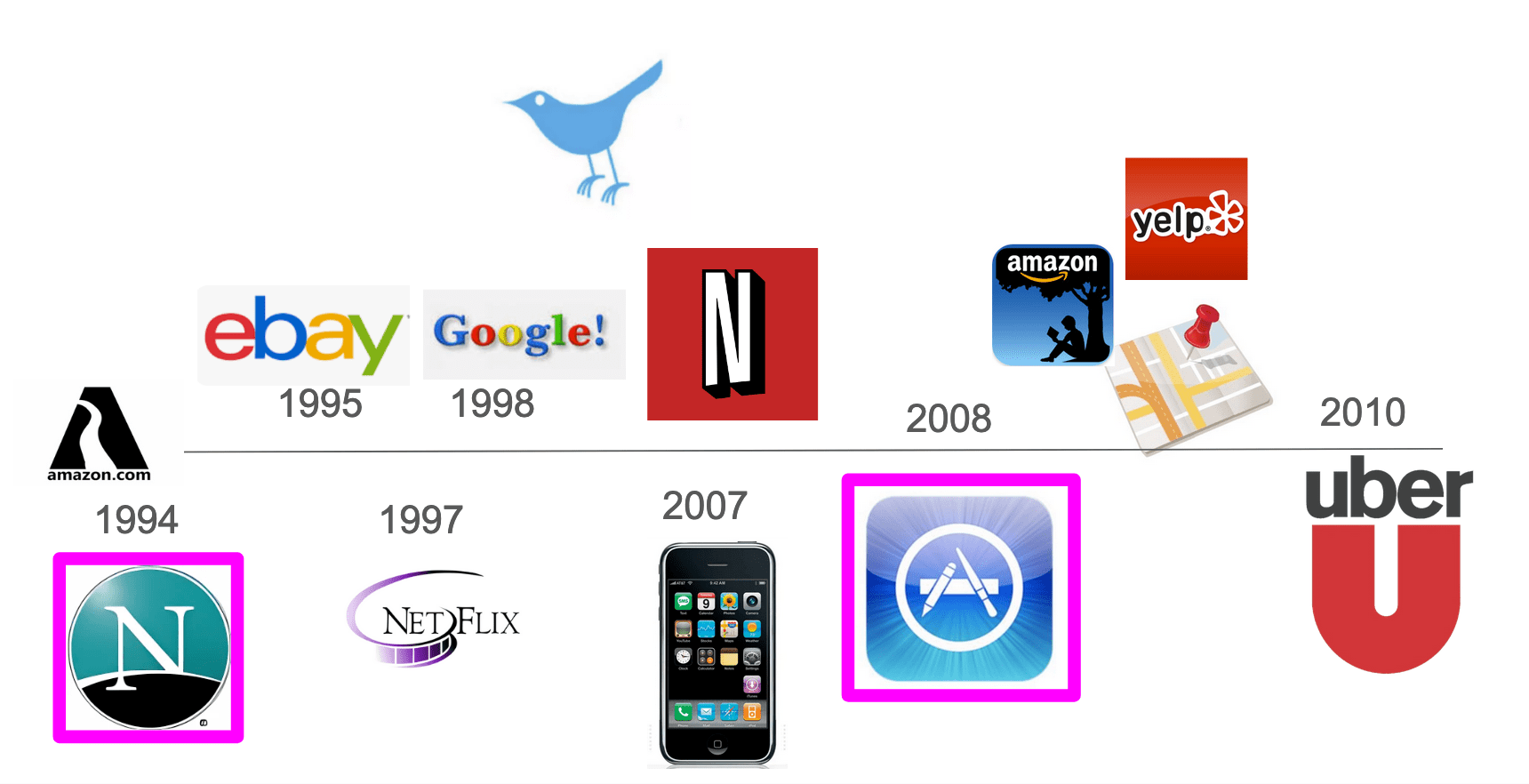
They also included Yelp, notably, an app with a garage dude story. Supposedly Jeremy Stoppelman, the wealthy and well-connected Paypal alum, caught the flu and couldn’t find a doctor to visit in San Francisco, and built Yelp for himself, and boom: success and riches. In 2010 we get the founding of Uber, supposedly the brainchild of rich tech alums Travis Kalanick and Garrett Camp after they couldn’t catch a cab in Paris during the LeWeb conference.
I find these anecdotal stories particularly illustrative of the privilege point. Imagine having the resources to be like “Man, catching that cab was annoying. Lemme just quit my current life trajectory and play with the idea of spinning up a whole company to make sure I don’t ever have to do that again. It’s okay if it fails, because I have the cushion to withstand that failure.” Superhuman vision didn’t catalyze this: it’s not like masses of sheeple relish in the experience of catching a cab and couldn’t describe a theoretical better option if they tried. It’s that realizing such a thing requires availability of copious investment capital in the face of non-negligible risk. People who can pursue this kind of thing are either previous-tech-exit-rich or poised-to-convince-venture-capitalists-rich. Their stories are fun to tell and hear, but not practical mogul origin stories for the vast majority of tech workers.
Since then, the mobile market has matured: you don’t hear Stoppelman’s or Kalanick’s story among the top apps from 2022, which mostly include established tech products like Facebook owned by Meta, the Whatsapp messaging app owned by Meta, and the Instagram photo social media app…also owned by Meta. You’ve also got Sequoia-backed Tiktok in there and CapCut, an editing app directed at Tiktok users. These are companies known—nah, notorious—for a voraciously data-driven approach to product development. Smaller operations can still find their niche in mobile, but the platform market maturation process moves faster now.
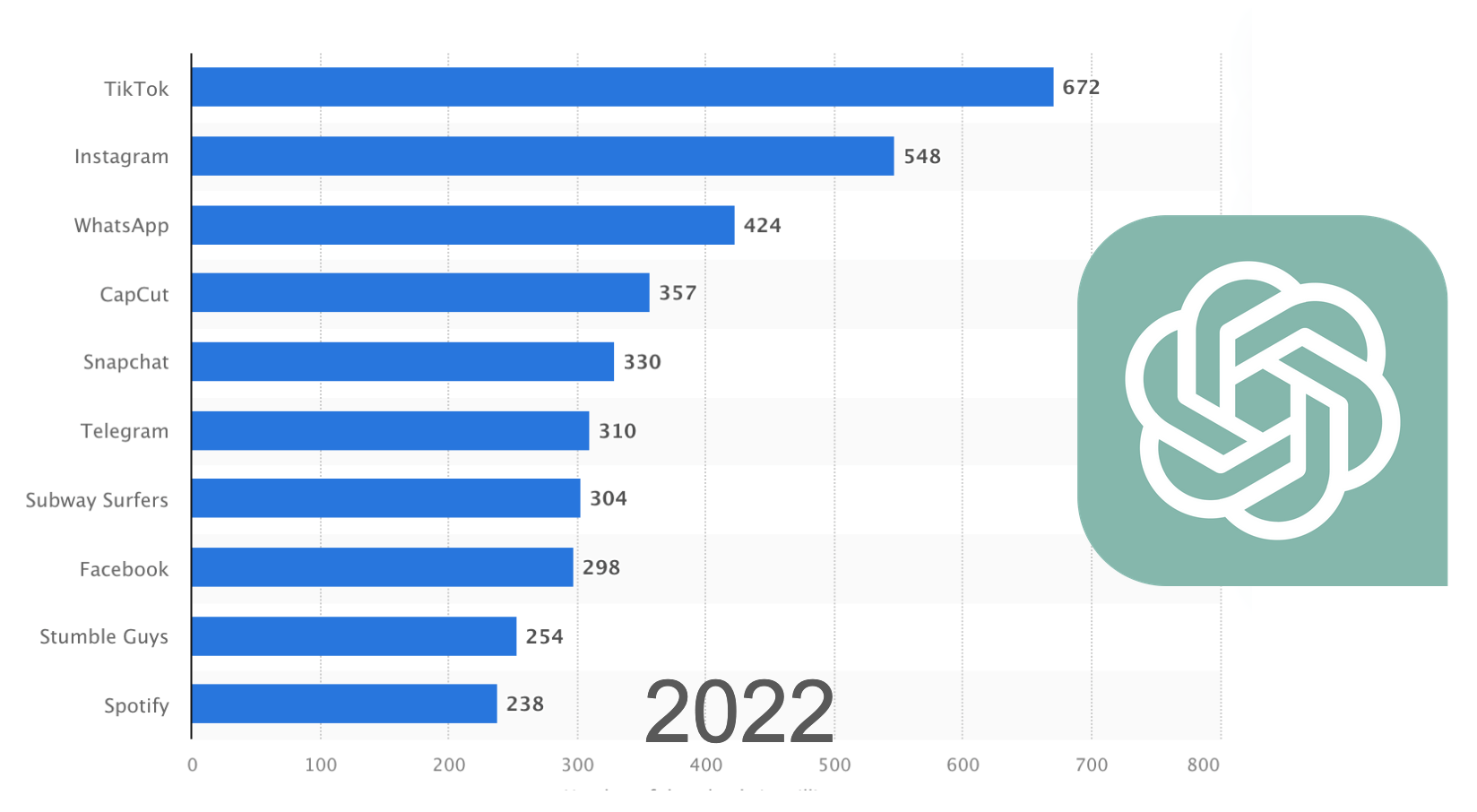
It moves faster because, with each platform, the folks who struck it rich in the first tech boom get further and further ahead. In the nineties, the Dorm Room Garage Dudes had an appreciable head start on relationships and resources to build the commercial web. But by the time the mobile platform came along, those same people had become billionaire tech moguls with cliques that garnered names like ‘The Paypal Mafia.’ This gave them an order of magnitude more opportunity to move first on mobile. Over time, that lead has continued to grow, and with it the time from market creation to market saturation has shortened. We don’t just have the opportunity to resort to marginally-centered product vision approaches. We must. Those are the accessible opportunities outside of major upfront capital, relationship, and research investments.
In 2022 we also got ChatGPT.
What is ChatGPT? It’s a product produced by a company, founded with heavy involvement from some big known tech names, currently backed by Microsoft to the tune of ten billion USD. ChatGPT also depends on ten to fifteen years of research from Google and others on specifically how to build large language models.
We tend to think of ChatGPT, its competitor Google Gemini, and text-to-image generators like Dall-E, Midjourney, and Stable Diffusion as products. But I think there’s an argument to be made hat they operate more like platforms: a sea shift, this time not in the hardware, but in
the software available for the construction of an entire market right on top.
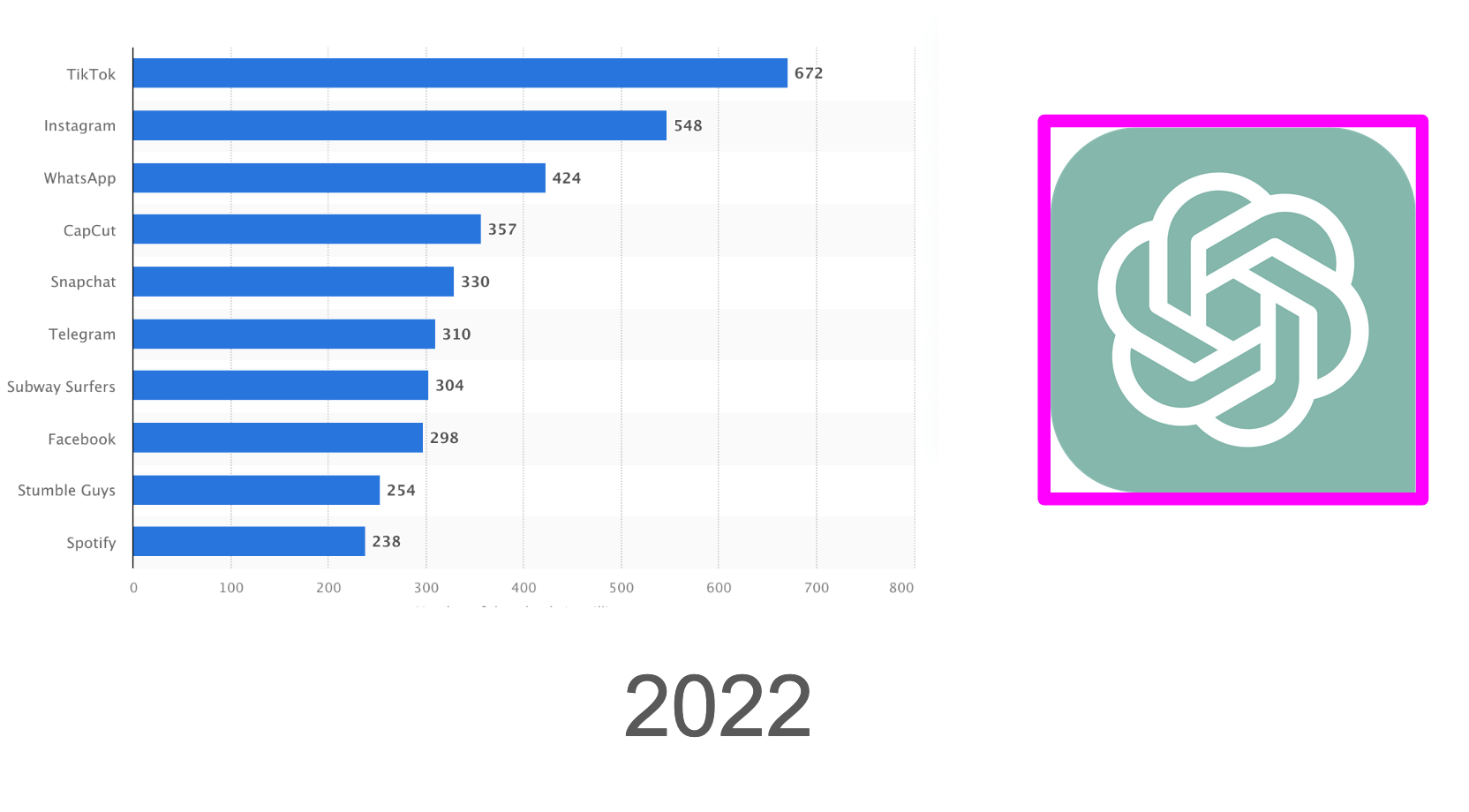
When we endeavor to think about the advent of generative models as a platform, we can structure an exploration of the tools we might build around the three generations we talked about. We’d be pretty close to the beginning of the platform market maturity cycle here: people are integrating with Generative models and trying to figure out how to make products with them. Every existing SaaS app’s landing page says “now with more AI!”
But if I’m right about the market maturation process speeding up as the lead of the first movers has increased, then that means the easy opportunities to build on top of this platform have gone.
First, we’ve got the open season where the new platform removes a limitation that so much of the population faces that just about anything addressing that limitation could see a lot of traction. What broadly experienced limitations do generative models address right now?
A big one is quotidian prose generation: models trained on the essays, text, and answers of the internet can reproduce that structure with reasonable reliability. The models replicate patterns in language: they don’t regurgitate truth or produce knowledge.
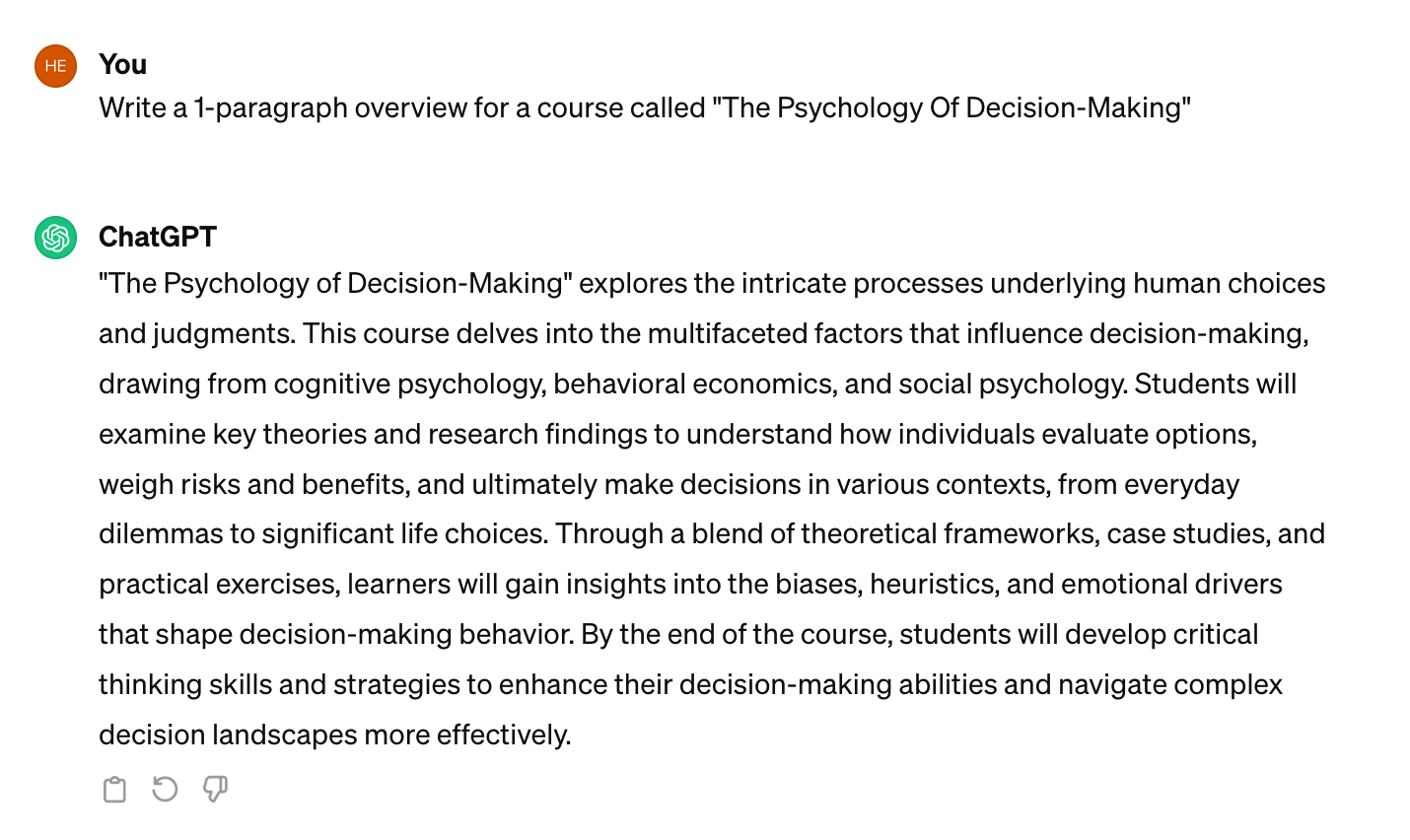
Here you can see I asked ChatGPT for a one paragraph overview of a course about the Psychology of Decision-Making. It can offer me a plausible course description, not because its understanding of pedagogy led it to this as the most appropriate description of such a class, but because it can replicate the language patterns of texts that discuss psychology.
But the replication of patterns in language, itself, removes a limitation. First, writing—even generic milquetoast writing of the kind produced by generative models—takes time to write by hand, and so they’ve seen massive adoption as a general-purpose templating tool for exactly that sort of milquetoast writing. Suppose you need to write a cover letter, and you’re experiencing a depresso espresso from losing your last job, managing your burnout, or living in an end-stage capitalist pandemic hellscape at the doorstep of a fascist takeover and a looming climate crisis. You can’t manage to get the dingdang thing done, and at this point you’re not trying to wow anyone; you just need something. ChatGPT can do that for you.
It can also draft you up a reference letter for a student or a colleague so you can skip the step where you look up the basic template and get right to filling in the vague and platitudinous central paragraphs with your actual anecdotes.
Or maybe your coworker is getting on your last nerve, but you can’t sound upset via email, so you need your thoughts translated into something more corporate acceptable. Or your boss’s boss’s boss’s boss has asked you, again, to justify precisely why you need time to update and maintain existing software, and “per my last email” has undergone too much semantic pejoration to actually say at work anymore, so you turn to the endlessly patient and deferential LLM.
But perhaps the most meaningful use case for a language replicator has to do with the specific language it works for: the language of the internet from whence its training data was scraped. As Timnit Gebru and her research colleagues correctly pointed out in the stochastic parrot paper, these LLMs train most and perform best on the linguistic tropes present in the language of the internet: well-off, well-connected, and in many datasets, largely anglophone. Folks who have written code for a decade but whose first language isn’t English can get help writing their cover letter to apply to a Californian software company and make it to the technical screen, where they get to showcase those coding skills.
We see a lot of the first movers fighting in this space right now—the folks with the capital to take the market for tools like these. I don’t know that it’s realistic to dream about a next-generation language replicator that beats the ones from Microsoft, Google, or Facebook-backed teams. Tech just isn’t anybody’s game like that; it hasn’t been for a long time.
But that doesn’t mean there aren’t plenty of other dreams still available. Besides the open season, we can leverage data—focus groups, aggregates, or even data collected or scraped from other products and companies—to find additional opportunities.
Within machine learning, the whole prospect of the thing being based on identifying patterns in data makes this an interesting one to consider. We’ve used classical machine learning models on tabular data for about a decade or so now to identify and leverage patterns in data:
- Figure out which customers have the highest estimated long term spending
prospects based on historical data, and pay the most attention to them. - Identify based on search habits at which point someone is ready to buy a
plane ticket, and gouge them at that point.
So on and so forth.
Generative machine learning focuses on identifying patterns in data precisely to replicate them; make text that sounds like a human might have written it. Generate images that look like the images a human might actually annotate with a text phrase like this one, or generate the text phrase given the image.
Replicating a pattern convincingly isn’t the same as factual accuracy, and we’ve begun to see some of the places where the patterns don’t include quite enough information to produce accurate results. People love the fingers one:
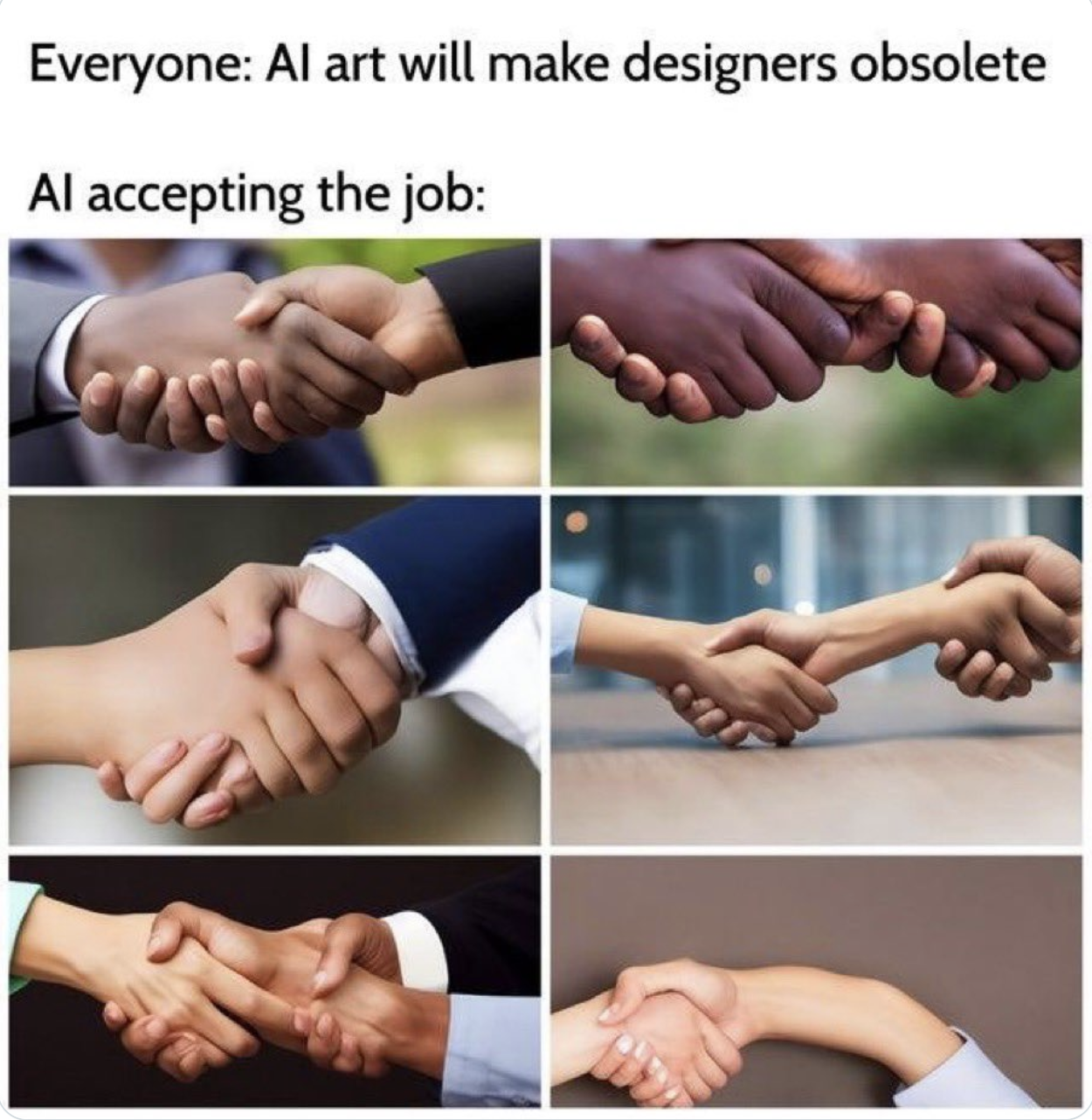
But some of the most both accessible and impactful examples come from professions that succeed and fail by their precise use of the appropriate proper nouns in the appropriate places. Proper nouns like the names of cases that a lawyer might try to use as legal precedent for a ruling they’re trying to get for a client. One of the thorny details of practicing law is that the cases you cite are supposed to exist.
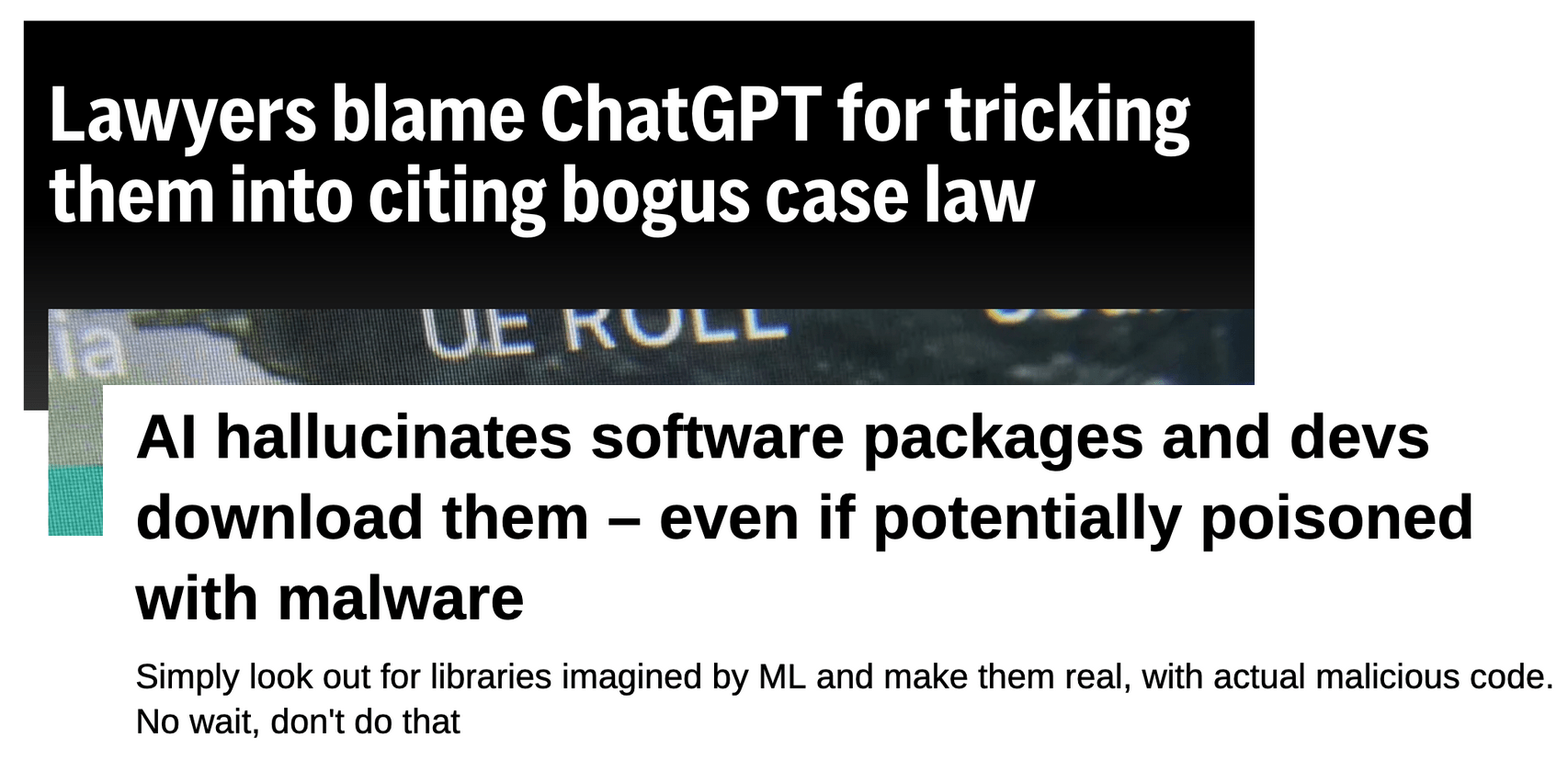
We face similar challenges in software engineering. If you’re trying to find a library in your programming language of choice to handle an integration, or cryptography, or any number of other tasks, best generally if it exists. Though, if you ask a chatbot for a library recommendation and it recommends you a cryptography library that doesn’t exist, that’s not the worst case scenario. Worse might be a library that does exist because a hacker asked the bot enough questions to understand what package names it would hallucinate, then built those packages with malware in them.
There’s a reason this happens. Replication of language patterns depends on identifying what words are used when. How is that done? Through context—the surrounding words, in particular the preceding words, during language generation. Part of speech words have strong relationships with context. After that, nouns, verbs, and adjectives that we use often in specific scenarios. You know what’s low on the list? Proper nouns, because they’re contextually interchangeable. We’d talk about or refer to any piece of case law, or any software library, with very similar language. So a language generator can easily put something there that sounds right within the context, but which just isn’t real.
I don’t think citing real case law or using real software packages is going to become less important, so the problem presents a demand for ways to identify what’s true. People regularly see a convincing language pattern in an LLM response and confuse it for truth.
We laugh at headlines like “Lawyers blame ChatGPT for tricking them into citing bogus case law” because they make a chatbot sound like some sort of duplicitous snake in the garden of Eden. But the claim, according to the firm that employed bogus-case-law-citer Steven Schwartz, is that Schwartz thought ChatGPT worked like a normal search engine. He figured he couldn’t verify the outputs merely because ChatGPT somehow had access to case law that he couldn’t access.
Now, If I wanted off the hook for something this embarrassing, the lie I’d tell would sound like this. I believe them, though. I believe them because I have mistakenly made myself the bearer of bad news on multiple occasions to professional software engineers who made a similar mistake. When ChatGPT says “I ran this code for you and got this result,” followed by a result that could not possibly follow from the code it’s talking about, it’s not because ChatGPT has some secret cutting-edge compiler hidden under the hood somewhere. What it’s doing is repeating the pattern it saw online in which StackOverflow people responded to questions with the words “I ran this code for you.” I had a man break down on me in a discord channel over this while I was trying to go get ice cream. I almost felt too guilty to eat the stuff.
False information isn’t a new problem; actual human beings have been bullshitting on the internet since the minute they got keyboards. Ebay and similar peer-to-peer sites implemented customer and then seller reviews as a means of popular verification. Google made its hay in the late ‘90s and early 2000s in search algorithms doing the same thing: attempting to weight the reputable sources more heavily, in part, based on how many other sites linked to them. WIkipedia has a whole editor system focused on preserving the site’s populist roots while maintaining the information’s accuracy.
When the fake review market came to game the customer reviews and search engine optimization came to game the search algorithms, new verification procedures emerged. In 2023 Mozilla purchased a company called Fakespot dedicated to using deep learning models to identify fake product reviews. Could popular verification, link tracing, communal editing, or automated classification strategies play a role in separating real and fake in generated text?
Today, researchers try to validate information out of LLMs by various means, or have models identify their sources, but it’s nowhere near a solved problem. Would fine tuning a general LLM on case law fix this? Or forcing it to check proper nouns against a registry? Or coming up with a different schema for precise professions like these entirely? I think there are a lot of questions to answer here, and tools that we don’t have yet.
Here’s a different question: could we systematically leverage these systems for building the actual understanding the systems lack? I don’t mean getting the language replicators to understand things. I mean using the language replicators to remove barriers to help human beings understand things. We’ve got plenty of data to suggest that it’s not just the machines who could use a little more understanding. What tools could we build to realize that opportunity?
In other words, what does data tell us about the opportunities that generative models reveal, and what do the models’ outputs tell us about the places where we can better understand and leverage the way we use them? I’ve offered some examples, but a data-driven line of inquiry can add quite a few items to the list of opportunities in tooling around generative models.
But I confess, it’s in the third generation zone that I find myself thinking the most. This is the generation of the platform maturity cycle in which the remaining opportunities come from centering precisely the marginal cases that the first two generations deliberately ignored, overlooked, missed, or cast aside. Though it requires perhaps the most creativity to consider, it’s also, I think, where the biggest innovations come from.
We understand that text-to-image generators train on massive web scrapes including the work of independent creators, and that those independent creators now feel deterred from posting their original work online. As we discussed before, the results from these generators are global averages: amalgams of what already exists. They can’t push boundaries in the creative direction: they can only mimic what they’ve seen artists do. So how do we go about preventing creative stagnation? Do we find ways to enforce copyright on the images themselves—introduce pixel-level adjustments to image data such that a model trained on that data performs poorly on production data? This is called “model poisoning.” Or maybe we change the value and the role of derivative work vs work with a specific voice.
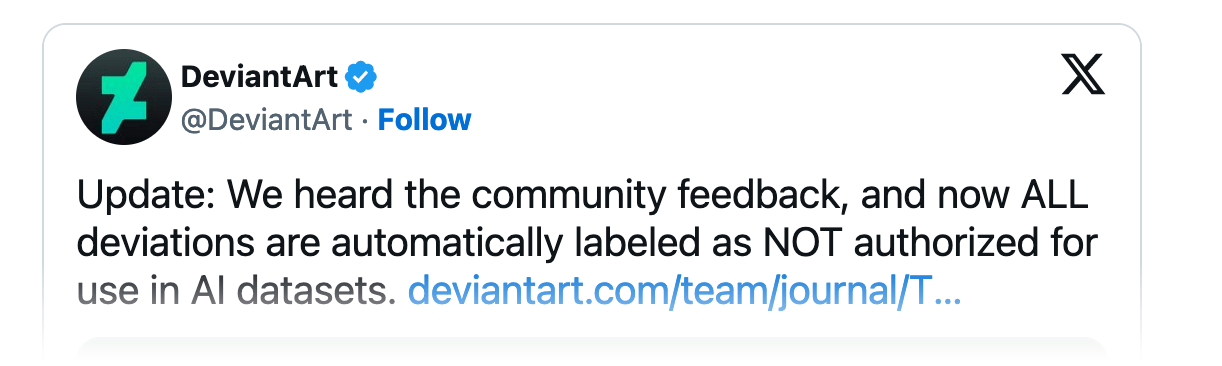
Similarly, we’ve seen the code these things write. If people copypasta that into their code bases for long enough, those code bases will end up becoming un-navigable. How do we prevent that? Do we need to worry about preventing that, if spinning up a new code base from scratch later might just take a few prompts? Do you think we’re about to be in a world of legacy hellscapes, or a world of constant rewrites? And how would we prepare for either future?
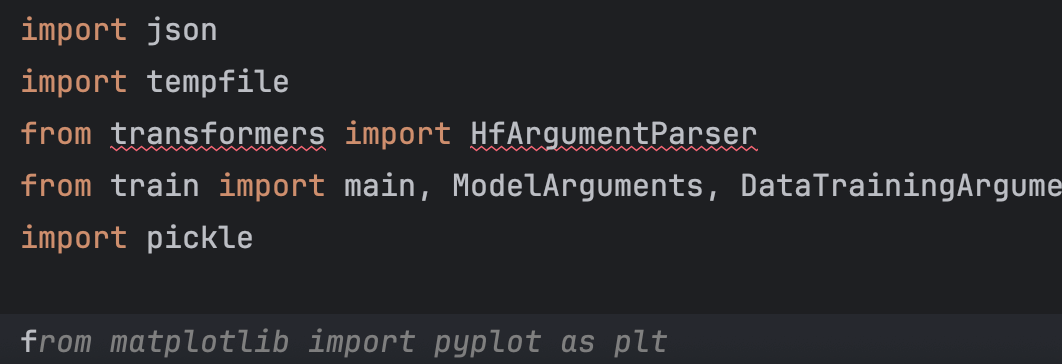
AI companies crow about their goal to build products that replicate all economically valuable human labor. What does “economically valuable” mean? What does that goal cast aside about the value of human labor? Labor history has a lot to say about what we count as “work;” perhaps even more about what we count as “compensatable work.” Which of the gaps there—between whatever Sam Altman meant by “economically valuable” and the actual sum of the labor to which the human species commits—can we find ways to serve?
In addition to those gaps, we have the gaps in social participation generated by inaccessible systems. What might we do about those? Improving human computer interaction for folks with motor impairments led to the screens of modern smartphones. Many of the world’s favorite “productivity hacks” grew from productionizations of coping mechanisms for folks with executive function disorders. What additional opportunities for innovation could we uncover by focusing on technology’s accessibility?
Accessibility innovation allows us to combat ableism, but that’s not the only frontier it pushes. Tech isn’t built to address the needs of disenfranchised single working mothers, or formerly incarcerated people, or trans youth. Those folks have vision that mainstream tech doesn’t have; can’t have. What products would these people spearhead, given the opportunity?
I don’t think the Paypal Mafia building for themselves can independently launch us into the future we want. I think they can rapidly saturate any market opened by a new tech platform. As a result, first-generation and even second-generation product ideas are done to death almost as soon as the platform becomes available. The surest, and maybe the only, pathways to innovation in a saturated market require a focus on the marginal cases. Who can best identify and solve for these cases? Often, it’s the people for whom the status quo works the least well—whose existence doesn’t even factor into status quo decision-making.
To the extent that the availability of generative models constitutes a new platform, that framework applies the same way it applied for the mobile platform and the consumer web. The perspectives, lived experiences, and contributions that would transition AI products from “expensive skeuomorph” to “meaningful innovation” won’t, and can’t, come from tech’s noveau riche. For that transition to materialize, the execution support available from the Paypal Mafia will have to go looking for who it has left behind.
I’m not holding my breath.
Footnotes
- For this post I’m, again, setting aside the fact that the term “artificial intelligence” is seventy years old, originally coined to describe any variety of computer-assisted decision making, and a superset of machine learning techniques—not a subset of it encompassing three particular consumer-facing products that rose to popularity in like 2021. I’m going to use the term the way journalists, executives, and product managers currently use it: as a stand-in for massive generative models specifically. I hate it, but I see the writing on the wall. ↩︎
- I’m starting this count at Tom Kilburn’s calculation of the highest factor of the integer 2^18 on the Manchester Baby computer on 21 June, 1948. That calculation by itself absolutely predates “commercial software,” but I don’t have the energy for 300 Gravatars in the comments section claiming I shortchanged the length of commercial computing history. ↩︎
- Not so much O’Reilly as smaller companies that trail and copy O’Reilly. ↩︎
- A this point we’re firmly in speculative territory, of course. I also don’t feel like getting sued or fired, so I’m committed to avoiding gratuitous messiness. But it doesn’t escape my notice that the past half decade for Google has included some metric selections that might not have exactly have encouraged product quality. I’m sure a subset of Googlers would also insist that OpenAI harbors fewer qualms than Google does about ignoring intellectual property rights to harvest training data. I do think that Google’s not-exactly-angelic history on the data privacy and multidecadal accumulated data from its enormous constituency make that a tough case to cleanly win, but I’m committed to fairness in the absence of certainty, so I’m mentioning it. ↩︎
If you liked this piece, you might also like:
How does AI impact my job as a programmer?
How do we evaluate people for their technical leadership?