On Friday we got the U.S. labour market report for July. Employment growth and wage growth continue to decelerate, and most notably, the unemployment rate rose to 4.3% in July, up from 4.1% in June.
This increase has triggered a “recession alarm” — at least if one subscribes to the so-called Sahm Rule.
Understanding the Sahm Rule
The Sahm Rule, devised by American economist Claudia Sahm, provides a strong indication of whether the U.S. economy is heading into a recession. It posits that if the three-month moving average of the unemployment rate rises by 0.5 percentage points or more above its lowest level within the past 12 months, it is a powerful signal that the economy is already in recession.
Although I am not necessarily of the opinion that the U.S. economy is in a recession at this moment, high-frequency growth indicators suggest that GDP growth in the U.S. is currently around 2-2.5%.
This implies that unemployment has been “too low” relative to the so-called structural unemployment (sometimes referred to as NAIRU), and a natural and necessary adjustment is taking place. I estimate that NAIRU is around 4.0-4.5%.
The Sahm Rule Triggered
Unemployment is indeed rising, as shown in the graph below, and the Sahm Rule has thus been “triggered”. The three-month moving average (the red line) is now precisely 0.5 percentage points above the lowest point over the last 12 months (the green line).
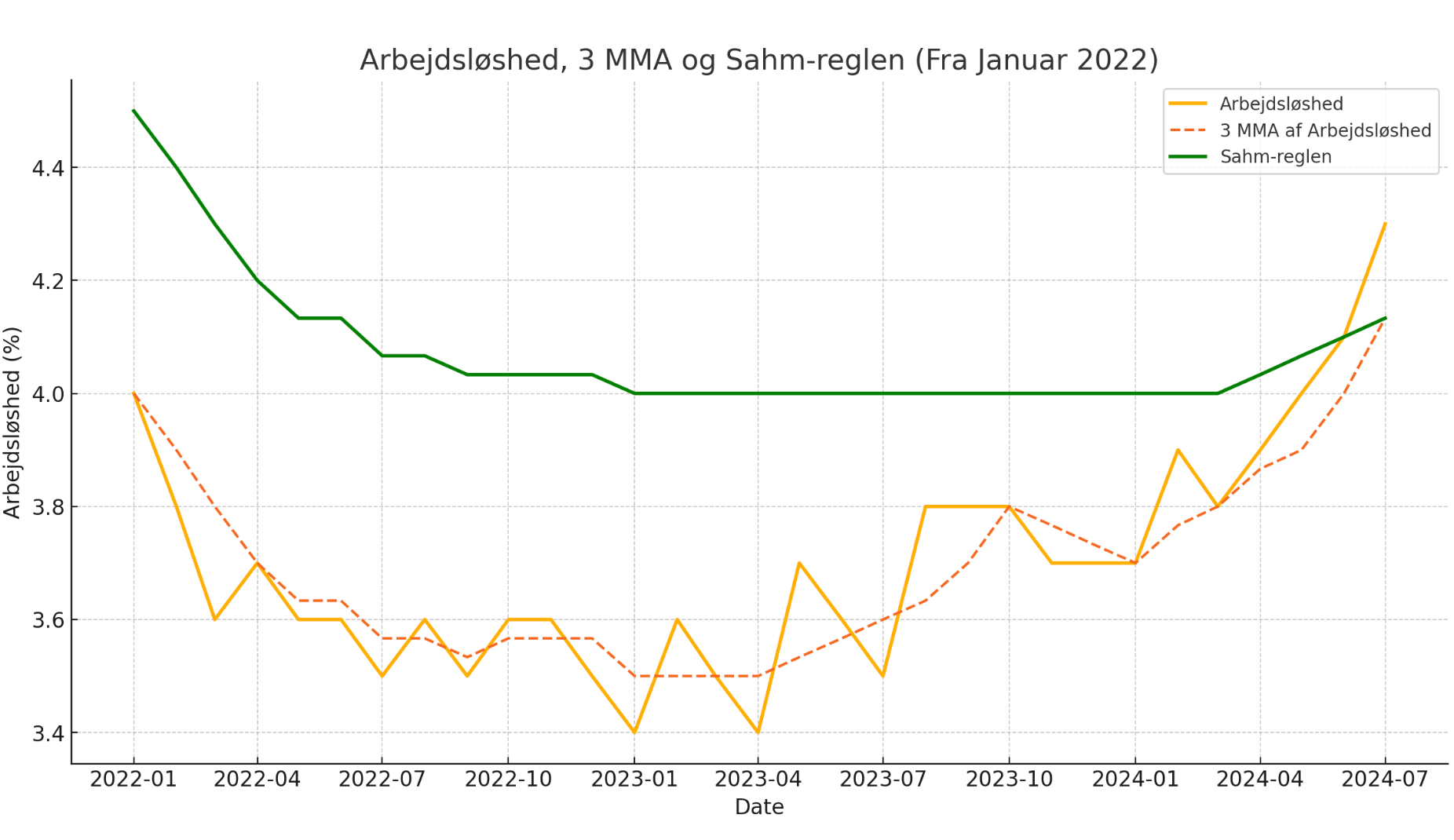
While I am sceptical that the US economy is actually in recession now, it is nonetheless clear that the US labour market has been cooling. At the same time, it is also clear that historically, the Fed has been too slow in changing direction when warranted and thereby has effectively triggered recessions by inappropriately changing monetary conditions when needed.
However, the purpose of this blog post is to investigate whether the fact that the Sahm Rule has been triggered tells us anything about the future or not.
Therefore, I have looked at all the occasions since 1960 when the Sahm Rule has been triggered and examined unemployment, inflation and the Fed funds rate following the triggering of the Sahm Rule.
The following episodes have been identified using the real-time Sahm Rule indicator:
- August 1960 to October 1961
- February 1970 to October 1971
- July 1974 to January 1976
- April 1980 to April 1981
- November 1981 to June 1983
- August 1990 to July 1991
- July 2001 to September 2003
- February 2008 to September 2009
- April 2020 to May 2020
I now use these dates to identify the beginning of a recessionary episode. These more or less correspond to the dates identified by the NBER, for example.
In the graphs below, we use these starting dates as period “zero” and look at 36 months prior and 36 months after the “trigger time” for unemployment, inflation and the fed funds rate.
We start with unemployment.
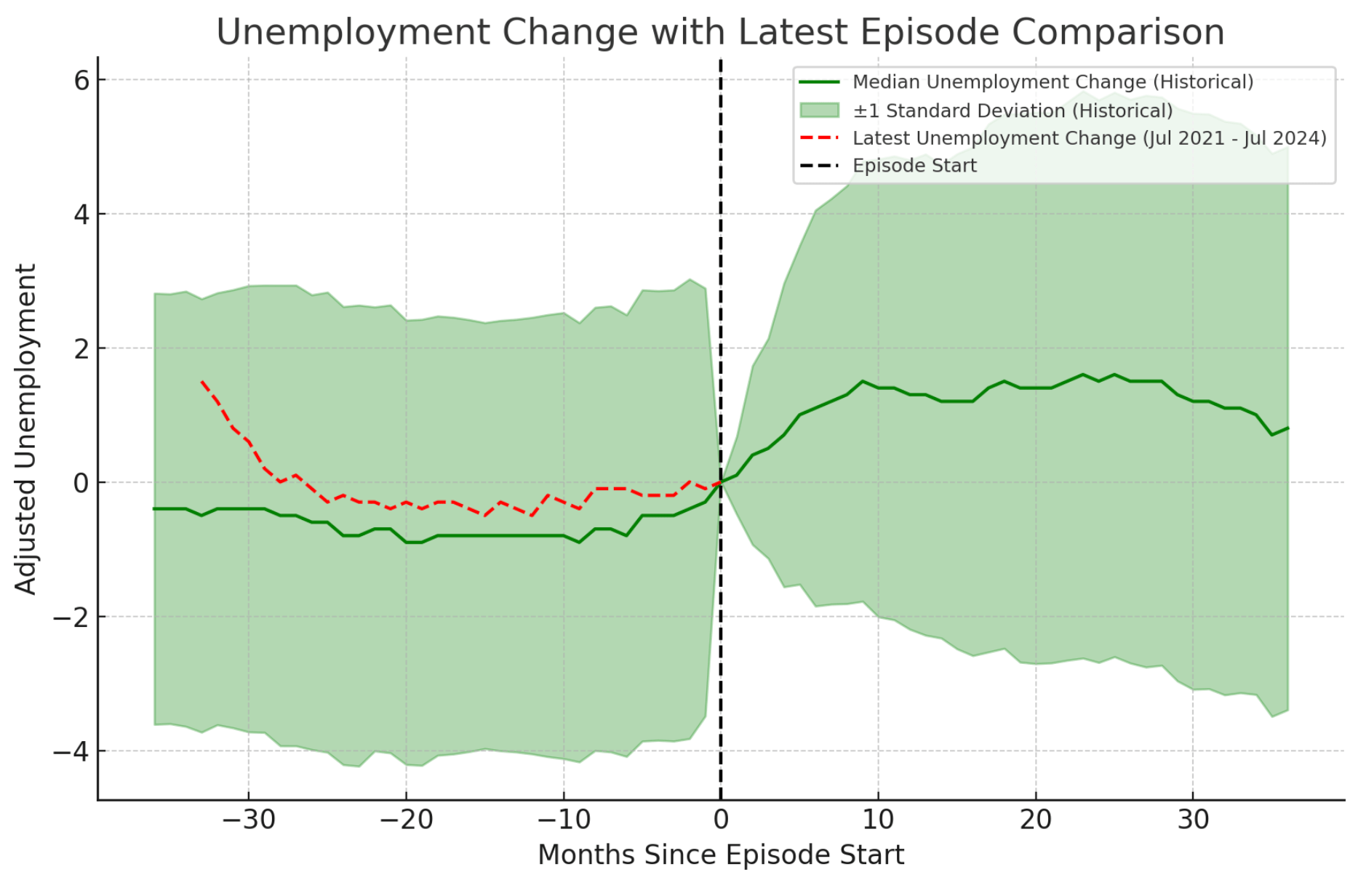
We start with unemployment.
If we look at the median across the historical episodes, then we see a gradual but moderate increase in unemployment prior to the “trigger date” (time zero), whereafter the increase in unemployment seems to accelerate. However, we also see that this pattern is far from clear as the “green band” is very wide – both prior to and after the trigger date. The band is +/- 1 standard deviation from the historical median.
But if we assume that the median tells us something about the future, then we should expect US unemployment to continue to increase going forward and at an accelerating rate. Judging from history, unemployment should increase by 1.5 percentage points over the next 10-12 months to just below 6%.
That said, it is also clear that the uptrend in US unemployment has been less strong this time around (the red line) than the historical median (the green line). To me, this is an indication that even though the Sahm Rule has been triggered, a recessionary shock really hasn’t hit (yet?), but that might of course change and this is a purely historical comparison.
Next up – inflation.
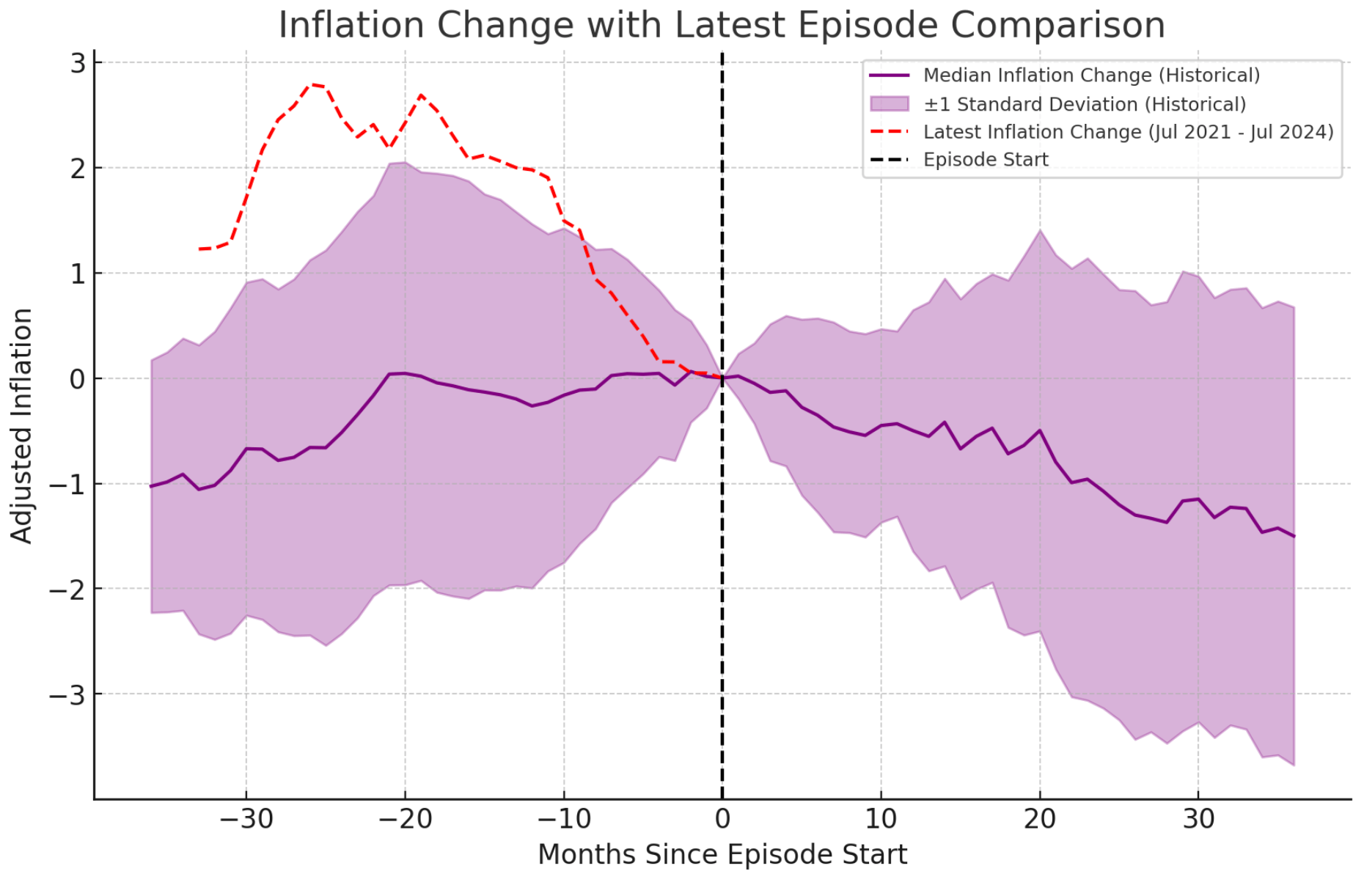
The graph shows a rather interesting pattern. Inflation tends to rise until around 20 months prior to the trigger date, whereafter it stabilises, but then around the trigger date, inflation starts to decline. Again, the “band” around the median is quite wide, but the “direction” seems to be clearer than for the unemployment data.
Again, this recent episode that really started with the lockdowns and the unprecedented easing of fiscal and monetary policy in the US in 2020-21 is somewhat different from the historical median. Hence, the increase in inflation was much stronger than historical, but it is in fact even more notable just how much inflation has come down over the past 2 years.
It is therefore also fair to question whether inflation will drop as much as the historical median. That being said, if we look at the first 15-20 months or so, then the historical indication is that inflation should drop by around 1 percentage points, which would mean inflation would drop just below 2%, which doesn’t seem all that far-fetched.
And finally, on to interest rates.
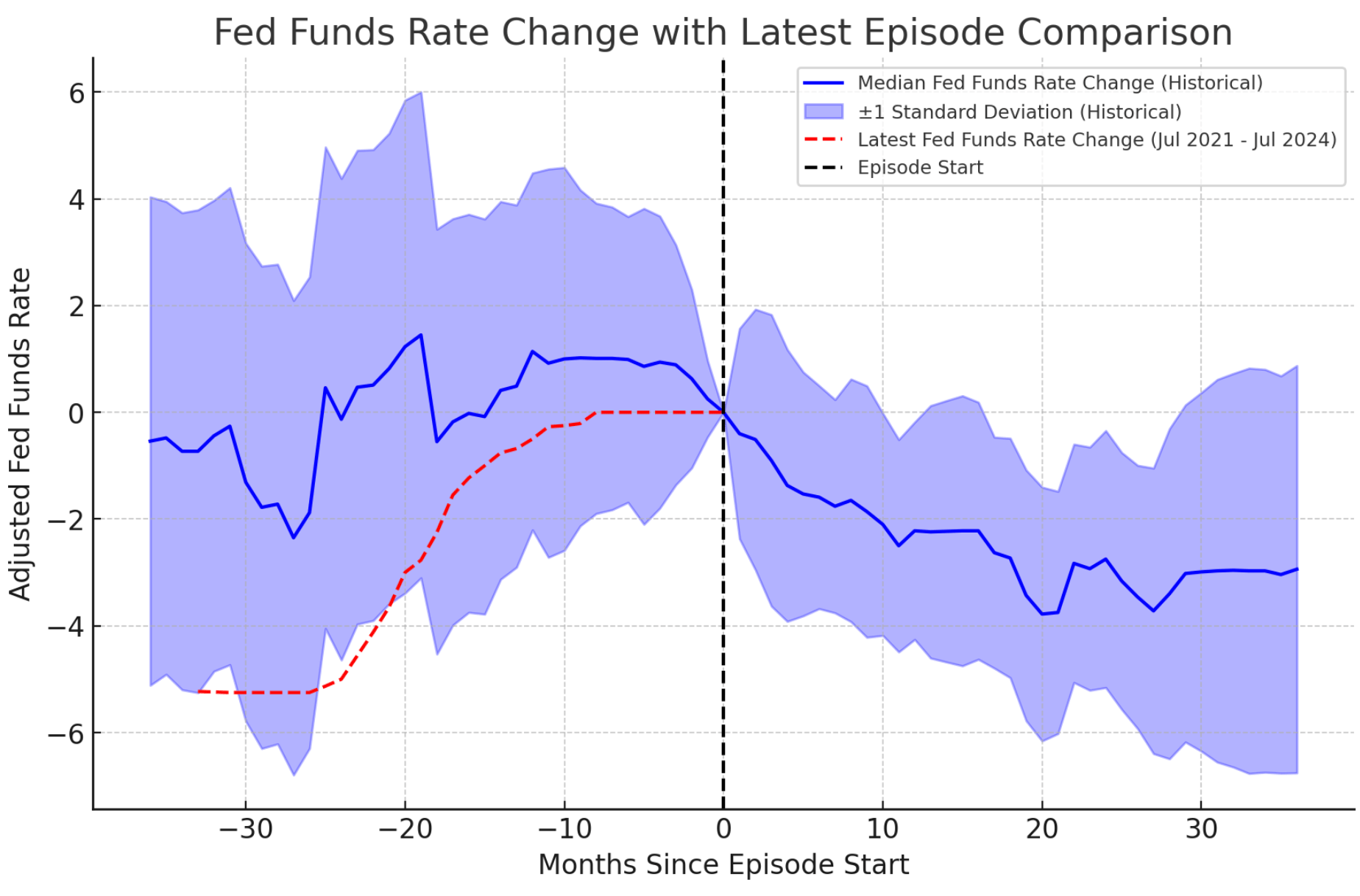
If we look at the Fed funds rate, the development in historical median is a bit messy, but the overall picture is of rising interest rates. This is not surprising as we have seen above rising inflation prior to the “trigger date”. So what we get the indication of is rising inflation followed by interest rate hikes from the Federal Reserve followed by a period where unemployment gradually rises and inflation starts to stabilise.
However, at some point the Fed “overdoes” it in terms of monetary tightening, and this triggers the recession and a sharper increase in unemployment.
It historically has taken time for the Fed to realise its mistake, but at some point quite close to the “trigger date”, the Fed does a U-turn and starts cutting interest rates as we all quite clearly see from the graph above.
In fact, the pattern is a bit clearer for interest rates than for unemployment and inflation, judging from the standard deviation band which is narrower for interest rates than for unemployment and inflation.
What we also see is that the cutting cycle is actually somewhat front-loaded. It might be that the Fed has taken too long to start cutting rates, but once it initiates its cutting cycle, the cuts come pretty fast. In fact, within the first year after the trigger date, the Fed historically has cut the fed funds rate by around 2 percentage points.
In the present situation, that would imply that the Fed would cut the Fed funds rates to 3.25-3.50%. This could seem like a lot, but in fact it is not significantly more than priced by the markets.
Furthermore, if we use the theoretical Mankiw rule that I discussed in my previous post (Eeny, Meeny, Miny, Mankiw: The Surprisingly Accurate Way to Guess Fed Policy) and assume that unemployment, for example, increases from presently 4.3% to 5.8% and PCE core inflation drops from 2.6% to 1.8% over the next 12-18 months, then we should actually expect interest rates to drop by 3-3.5 percentage points – down to around 2%.
Hence, even though market pricing in relation to rate cuts has changed rather dramatically over the past week, the markets are still not priced for median historical recession.
Even though it is a pretty daring assessment to make, based on market pricing and the historical relationship between unemployment and the fed funds rates (for a given inflation outlook), I would estimate that markets are presently pricing US unemployment to rise to around 5.0%.
That surely would be seen as a recession, but in a historical perspective, it would be a quite mild recession.
Overall, if I should compare it to anything, I think this is quite similar to the relatively mild and short recession that also followed a tech-driven stock market boom and where the fed funds rates were at a similar level at the “trigger date” as presently is the case, but where also rates were cut relatively swiftly. However, at that time we also had a massive uncertainty shock as a consequence of the terror attacks against the US on 9/11 – on top of the recession that had already started. Hence, we effectively had a double-shock in 2000-1 and without 9/11, the cutting cycle would likely have ended earlier – meaning that the comparison would have been a total cutting cycle of around 3 percentage points.
The paradox here is that if the Fed takes too long to start cutting rates, it would cause a sharper increase in unemployment and in inflation, which in turn will necessitate an even stronger monetary policy response and deeper rate cuts.
Another policy mistake?
Former IMF chief economist Rudiger Dornbusch is famous for saying that the Fed usually murders the expansion before it gets a chance to die of old age. Our analysis above indicates why this is the case – the Fed simply takes too long turning around and historically has initiated the easing cycle too late.
The good news is, however, as long as the zero lower bound is not hit, the Fed normally corrects its mistake relatively fast, and I am even tempted to say that Fed-chairman Jerome Powell full-well understands this and has the means to ensure “Growth Forever” without a recession.
However, I don’t dare argue that – after all, this is exactly the argument Rudiger Dornbusch made in 1998 in an article with exactly that title – “Growth Forever” – in which he argued that the Fed had now learned how to avoid a recession. But the recession nonetheless came in 2000-1.
And I have long argued that for now, the Fed seems to have managed to engineer a “soft landing”, but I must also admit I am not fully convinced that the Fed will continue to manage well. Even though Powell now clearly has signalled that the Fed will soon start cutting rates, it is also important to understand that we are at a critical point where the Fed should be extremely focused on not getting behind the curve.
The US is still not in recession, but it is also the Fed’s job not to trigger a recession, and the track record is quite bad.
PS the best way to avoid remaking the mistakes of the past is to change the Fed’s policy framework to a market-based and truly forward-looking monetary policy regime.
PPS if you or Jerome Powell is looking for a easy way to calibrate rate policy have a look at my interest rate calculator here. The calculator is created with Claude 3.5 Sonnet.
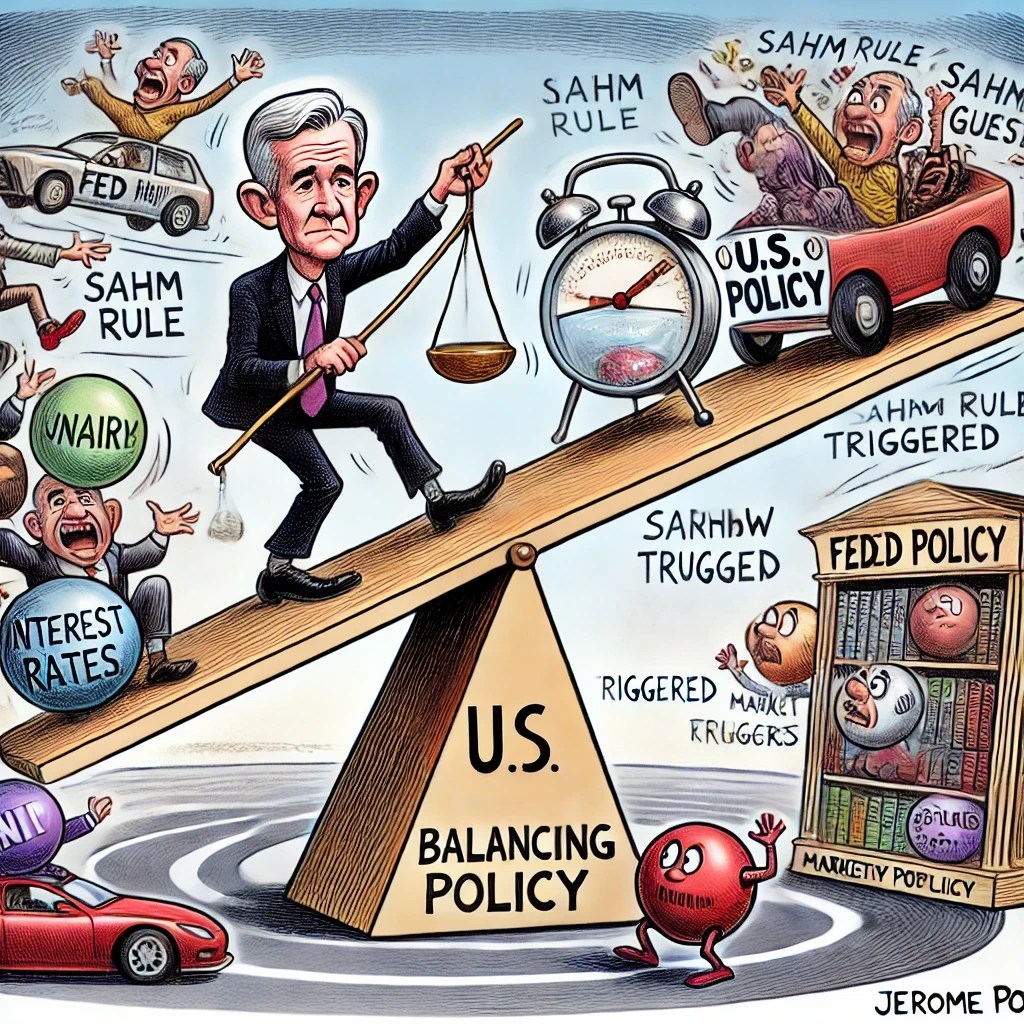
Note: Illustration created with ChatGPT 4o/Dall-E.
If you want to know more about my work on AI and data, then have a look at the website of PAICE — the AI and data consultancy I have co-founded.